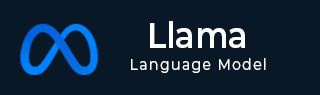
- Llama 教程
- Llama - 首页
- Llama - 环境设置
- Llama - 快速上手
- Llama - 数据准备
- Llama - 从零开始训练
- Llama 模型微调
- Llama - 模型性能评估
- Llama - 模型优化
- Llama 有用资源
- Llama - 快速指南
- Llama - 有用资源
- Llama - 讨论
Llama 数据准备
良好的数据准备对于训练任何高性能语言模型(例如 Llama)都至关重要。数据准备包括收集和清理数据、准备 Llama 可用的数据以及使用不同的数据预处理器。NLTK、spaCy 和 Hugging Face 分词器等工具共同帮助将数据准备好用于 Llama 的训练流程。一旦您了解了这些数据预处理阶段,您就能确保提高 Llama 模型的性能。
数据准备被认为是机器学习模型中最关键的阶段之一,尤其是在处理大型语言模型时。本章讨论如何准备可用于 Llama 的数据,并涵盖以下主题。
- 数据收集和清理
- 为 Llama 格式化数据
- 数据预处理中使用的工具
所有这些过程确保数据得到良好清理并进行适当的结构化,从而优化 Llama 的管道训练。
数据收集和清理
数据收集
与训练 Llama 等模型相关的最关键点是高质量的多样化数据。换句话说,运行语言模型时用于训练的主要文本数据来源是来自各种文本的片段,包括书籍、文章、博客文章、社交媒体内容、论坛和其他公开可用的文本数据。
使用 Python 抓取网站文本数据
import requests from bs4 import BeautifulSoup # URL to fetch data from url = 'https://tutorialspoint.com/Llama/index.htm' response = requests.get(url) soup = BeautifulSoup(response.text, 'html.parser') # Now, extract text data text_data = soup.get_text() # Now, save data to the file with open('raw_data.txt', 'w', encoding='utf-8') as file: file.write(text_data)
输出
运行脚本后,它会将抓取的文本保存到名为 raw_data.txt 的文件中,然后将原始文本清理成数据。
数据清理
原始数据充满了噪声,包括 HTML 标签、特殊字符和原始数据中不相关的数据,因此必须在呈现给 Llama 之前进行清理。数据清理可能包括:
- 删除 HTML 标签
- 特殊字符
- 大小写敏感性
- 分词
- 去除停用词
示例:使用 Python 预处理文本数据
import re from nltk.corpus import stopwords from nltk.tokenize import word_tokenize import nltk nltk.download('punkt') nltk.download('stopwords') # Load raw data with open('/raw_data.txt', 'r', encoding='utf-8') as file: text_data = file.read() # Clean HTML tags clean_data = re.sub(r'<.*?>', '', text_data) # Clean special characters clean_data = re.sub(r'[^A-Za-z0-9\\\\\\s]', '', clean_data) # Split text into tokens tokens = word_tokenize(clean_data) stop_words = set(stopwords.words('english')) # Filter out stop words from tokens filtered_tokens = [w for w in tokens if not w.lower() in stop_words] # Save cleaned data with open('cleaned_data.txt', 'w', encoding='utf-8') as file: file.write(' '.join(filtered_tokens)) print("Data cleaned and saved to cleaned_data.txt")
输出
Data cleaned and saved to cleaned_data.txt
清理后的数据将保存到 cleaned_data.txt。该文件现在包含分词和清理后的数据,已准备好用于 Llama 的进一步格式化和预处理。
预处理数据以与 Llama 配合使用
Llama 需要预先结构化的输入数据进行训练。数据应该被分词,也可以根据其将要使用的架构转换为 JSON 或 CSV 等格式。
文本分词
文本分词是将句子分成较小部分(通常是单词或子词)的行为,以便 Llama 可以处理它们。您可以使用预构建的库,包括 Hugging Face 的分词器库。
from transformers import LlamaTokenizer # token = "your_token" # Sample sentence text = "Llama is an innovative language model." #Load Llama tokenizer tokenizer = LlamaTokenizer.from_pretrained("meta-llama/Llama-2-7b-hf", token=token) #Tokenize encoded_input = tokenizer(text) print("Original Text:", text) print("Tokenized Output:", encoded_input)
输出
Original Text: Llama is an innovative language model. Tokenized Output: {'input_ids': [1, 365, 29880, 3304, 338, 385, 24233, 1230, 4086, 1904, 29889], 'attention_mask': [1, 1, 1, 1, 1, 1, 1, 1, 1, 1, 1]}
将数据转换为 JSON 格式
JSON 格式与 Llama 相关,因为它以结构化的方式存储文本数据。
import json # Data structure data = { "id": "1", "text": "Llama is a powerful language model for AI research." } # Save data as JSON with open('formatted_data.json', 'w', encoding='utf-8') as json_file: json.dump(data, json_file, indent=4) print("Data formatted and saved to formatted_data.json")
输出
Data formatted and saved to formatted_data.json
程序将打印一个名为 formatted_data.json 的文件,其中包含 JSON 格式的格式化文本数据。
数据预处理工具
数据清理、分词和格式化工具适用于 Llama。最常用的工具组是使用 Python 库、文本处理框架和命令找到的。以下是 Llama 数据准备中一些广泛应用的工具列表。
1. NLTK (自然语言工具包)
最著名的自然语言处理库是 NLTK。该库支持的功能包括文本数据的清理、分词和词干提取。
示例:使用 NLTK 去除停用词
import nltk from nltk.corpus import stopwords nltk.download('punkt') nltk.download('stopwords') # Test Data text = "This is a simple sentence with stopwords." # Tokenization words = nltk.word_tokenize(text) # Stopwords stop_words = set(stopwords.words('english')) filtered_text = [w for w in words if not w.lower() in stop_words] # This line is added to filter the words and assign to the variable print("Original Text:", text) print("Filtered Text:", filtered_text)
输出
Original Text: This is a simple sentence with stopwords. Filtered Text: ['simple', 'sentence', 'stopwords', '.']
2. spaCy
另一个为数据预处理设计的、高级的库。它也快速、高效,并且构建用于 NLP 任务的实际应用。
示例:使用 spaCy 进行分词
import spacy # Load spaCy model nlp = spacy.load("en_core_web_sm") # Sample sentence text = "Llama is an innovative language model." # Process the text doc = nlp(text) # Tokenize tokens = [token.text for token in doc] print("Tokens:", tokens)
输出
Tokens: ['Llama', 'is', 'an', 'innovative', 'language', 'model', '.']
3. Hugging Face 分词器
Hugging Face 提供了一些高性能的分词器,这些分词器主要用于训练语言模型,而不是 Llama 本身。
示例:使用 Hugging Face 分词器
from transformers import AutoTokenizer token = "your_token" # Sample sentence text = "Llama is an innovative language model." #Load Llama tokenizer tokenizer = AutoTokenizer.from_pretrained('meta-llama/Llama-2-7b-hf', token=token) #Tokenize encoded_input = tokenizer(text) print("Original Text:", text) print("Tokenized Output:", encoded_input)
输出
Original Text: Llama is an innovative language model. Tokenized Output: {'input_ids': [1, 365, 29880, 3304, 338, 385, 24233, 1230, 4086, 1904, 29889], 'attention_mask': [1, 1, 1, 1, 1, 1, 1, 1, 1, 1, 1]}
4. Pandas 用于数据格式化
在处理结构化数据时使用。您可以使用 Pandas 将数据格式化为 CSV 或 JSON,然后再将其传递给 Llama。
import pandas as pd # Data structure data = { "id": "1", "text": "Llama is a powerful language model for AI research." } # Create DataFrame with an explicit index df = pd.DataFrame([data], index=[0]) # Creating a list of dictionary and passing an index [0] # Save DataFrame to CSV df.to_csv('formatted_data.csv', index=False) print("Data saved to formatted_data.csv")
输出
Data saved to formatted_data.csv
格式化的文本数据将保存在 CSV 文件 formatted_data.csv 中。