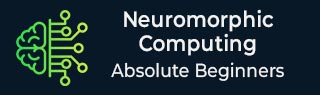
- 神经形态计算的应用
- 神经形态计算 - 边缘计算
- 神经形态计算 - 物联网 (IoT)
- 神经形态计算 - 机器人技术
- 神经形态计算 - 自动系统
- 神经形态计算 - AI 和 ML
- 神经形态计算 - 认知计算
- 神经形态计算资源
- 神经形态计算 - 有用资源
- 神经形态计算 - 讨论
神经形态计算 - 编程范式
在神经形态计算中,几种编程范式已被用于创建可靠的系统,每种范式都有其自身的特性和用途。在本节中,我们将详细讨论事件驱动编程、数据流编程和混合方法等范例,以及它们在神经形态计算中的示例和应用。
事件驱动编程
在事件驱动编程中,程序的流程由事件决定,例如用户交互或传感器输入。事件驱动模型能够模拟脉冲神经元,其中神经元只有在接收到脉冲时才处理信息。这种方法确保了高效的资源利用和实时处理,使其适用于机器人技术和传感器网络中的应用。
事件驱动编程示例 (Python)
下面的 Python 代码演示了一个简单的脉冲神经元的事件驱动模型,当其膜电位(神经元内部的电荷)超过阈值时,该神经元会触发一个事件。
class SpikingNeuron: def __init__(self, threshold=1.0): self.membrane_potential = 0.0 self.threshold = threshold def receive_input(self, input_current): self.membrane_potential += input_current if self.membrane_potential >= self.threshold: self.fire() def fire(self): print("Neuron fired!") self.membrane_potential = 0.0 # Reset after firing # Example usage neuron = SpikingNeuron() neuron.receive_input(0.5) neuron.receive_input(0.6) # This should trigger a fire event
在这个例子中,`SpikingNeuron` 类模拟了一个简单的接收输入电流的神经元,并在膜电位超过指定阈值时触发事件。
数据流编程
在数据流编程范式中,程序的执行由数据的可用性决定。在神经形态计算中,这种范式可以将信息在神经元之间的流动建模为数据在网络中移动。数据流编程允许并行执行,使其能够高效地模拟大规模神经网络。
数据流编程示例 (Python)
以下示例演示了一种模拟温度传感器网络的数据流方法,该网络在温度读数可用时立即处理读数。
class Sensor: def __init__(self, sensor_id): self.sensor_id = sensor_id self.data = None def receive_data(self, data): self.data = data return self.process_data() def process_data(self): # If the temperature exceeds 30°C, raise an alert if self.data > 30: return f"Sensor {self.sensor_id}: Temperature exceeds threshold! ({self.data}°C)" else: return f"Sensor {self.sensor_id}: Temperature is normal. ({self.data}°C)" # Simulating a network of sensors temperature_readings = [25, 35, 28, 31, 29] sensors = [Sensor(sensor_id=i) for i in range(1, 6)] # Processing temperature data in parallel when it's available outputs = [sensor.receive_data(temp) for sensor, temp in zip(sensors, temperature_readings)] for output in outputs: print(output)
在这个例子中,每个传感器并行处理其温度读数,展示了数据流范式同时处理多个输入的能力。
广告