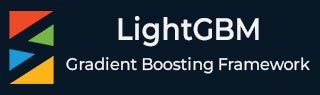
- LightGBM 教程
- LightGBM - 首页
- LightGBM - 概述
- LightGBM - 架构
- LightGBM - 安装
- LightGBM - 核心参数
- LightGBM - Boosting 算法
- LightGBM - 树生长策略
- LightGBM - 数据集结构
- LightGBM - 二分类
- LightGBM - 回归
- LightGBM - 排序
- LightGBM - Python 实现
- LightGBM - 参数调优
- LightGBM - 绘图功能
- LightGBM - 早停训练
- LightGBM - 特征交互约束
- LightGBM 与其他 Boosting 算法对比
- LightGBM 有用资源
- LightGBM - 有用资源
- LightGBM - 讨论
LightGBM - 早停训练
早停训练是一种方法,如果评估数据集上评估的评估指标在特定次数的循环后没有改善,我们就会停止训练。Lightgbm 的类似 sklearn 的估计器在 train() 和 fit() 方法中都有一个名为 early_stopping_rounds 的参数。此参数接受一个整数值,表示如果评估指标结果在一定轮数后没有改善,则应停止训练过程。
此参数接受一个整数值,表示如果评估指标结果在几轮后没有改善,则应终止训练过程。
因此请记住,这需要一个评估数据集才能工作,因为它依赖于针对评估数据集评估的评估指标结果。
示例
在加载波士顿房价数据集之前,我们将首先导入必要的库。从 1.2 版本开始,Scikit-Learn 中不再提供此数据集,因此我们将使用 sklearn.datasets.load_boston() 复制该特征。
from sklearn.model_selection import train_test_split X_train, X_test, Y_train, Y_test = train_test_split(boston.data, boston.target) print("Sizes of Train or Test Datasets : ", X_train.shape, X_test.shape, Y_train.shape, Y_test.shape) train_dataset = lgb.Dataset(X_train, Y_train, feature_name=boston.feature_names.tolist()) test_dataset = lgb.Dataset(X_test, Y_test, feature_name=boston.feature_names.tolist()) booster = lgb.train({"objective": "regression", "verbosity": -1, "metric": "rmse"}, train_set=train_dataset, valid_sets=(test_dataset,), early_stopping_rounds=5, num_boost_round=100) from sklearn.metrics import r2_score test_preds = booster.predict(X_test) train_preds = booster.predict(X_train) # Display the R2 scores in the console print("\nR2 Score on Test Set : %.2f"%r2_score(Y_test, test_preds)) print("R2 Score on Train Set : %.2f"%r2_score(Y_train, train_preds))
输出
这将产生以下结果
Sizes of Train or Test Datasets: (404, 13) (102, 13) (404,) (102,) [1] valid_0's rmse: 9.10722 Training until validation scores don't improve for 5 rounds [2] valid_0's rmse: 8.46389 [3] valid_0's rmse: 7.93394 [4] valid_0's rmse: 7.43812 [5] valid_0's rmse: 7.01845 [6] valid_0's rmse: 6.68186 [7] valid_0's rmse: 6.43834 [8] valid_0's rmse: 6.17357 [9] valid_0's rmse: 5.96725 [10] valid_0's rmse: 5.74169 [11] valid_0's rmse: 5.55389 [12] valid_0's rmse: 5.38595 [13] valid_0's rmse: 5.24832 [14] valid_0's rmse: 5.13373 [15] valid_0's rmse: 5.0457 [16] valid_0's rmse: 4.96688 [17] valid_0's rmse: 4.87874 [18] valid_0's rmse: 4.8246 [19] valid_0's rmse: 4.75342 [20] valid_0's rmse: 4.69854 Did not meet early stopping. Best iteration is: [20] valid_0's rmse: 4.69854 R2 Score on Test Set: 0.81 R2 Score on Train Set: 0.97
此程序将乳腺癌数据集分成两个部分,如训练和测试。它训练一个 LightGBM 模型来判断肿瘤是危险的还是无害的,因此如果性能没有改善,则提前停止。最后,它预测测试集和训练集的结果,并计算模型的准确率。
from sklearn.model_selection import train_test_split X_train, X_test, Y_train, Y_test = train_test_split(breast_cancer.data, breast_cancer.target) print("Sizes of Train or Test Datasets : ", X_train.shape, X_test.shape, Y_train.shape, Y_test.shape) booster = lgb.LGBMModel(objective="binary", n_estimators=100, metric="auc") booster.fit(X_train, Y_train, eval_set=[(X_test, Y_test),], early_stopping_rounds=3) from sklearn.metrics import accuracy_score test_preds = booster.predict(X_test) train_preds = booster.predict(X_train) test_preds = [1 if pred > 0.5 else 0 for pred in test_preds] train_preds = [1 if pred > 0.5 else 0 for pred in train_preds] # Display the accuracy results print("\nAccuracy Score on Test Set : %.2f"%accuracy_score(Y_test, test_preds)) print("Accuracy Score on Train Set : %.2f"%accuracy_score(Y_train, train_preds))
输出
这将导致以下结果
Sizes of Train or Test Datasets : (426, 30) (143, 30) (426,) (143,) [1] valid_0's auc: 0.986129 Training until validation scores don't improve for 3 rounds [2] valid_0's auc: 0.989355 [3] valid_0's auc: 0.988925 [4] valid_0's auc: 0.987097 [5] valid_0's auc: 0.990108 [6] valid_0's auc: 0.993011 [7] valid_0's auc: 0.993011 [8] valid_0's auc: 0.993441 [9] valid_0's auc: 0.993441 [10] valid_0's auc: 0.994194 [11] valid_0's auc: 0.994194 [12] valid_0's auc: 0.994194 [13] valid_0's auc: 0.994409 [14] valid_0's auc: 0.995914 [15] valid_0's auc: 0.996129 [16] valid_0's auc: 0.996989 [17] valid_0's auc: 0.996989 [18] valid_0's auc: 0.996344 [19] valid_0's auc: 0.997204 [20] valid_0's auc: 0.997419 [21] valid_0's auc: 0.997849 [22] valid_0's auc: 0.998065 [23] valid_0's auc: 0.997849 [24] valid_0's auc: 0.998065 [25] valid_0's auc: 0.997634 Early stopping, best iteration is: [22] valid_0's auc: 0.998065 Accuracy Score on Test Set : 0.97 Accuracy Score on Train Set : 0.98
如何通过“early_stopping()”回调提前停止训练?
LightGBM 实际上支持使用 early_stopping() 回调机制进行早停训练。我们可以将 early_stopping() 函数的轮数作为回调参数传递给 train()/fit() 方法。回调的使用如下所示:
from sklearn.model_selection import train_test_split X_train, X_test, Y_train, Y_test = train_test_split(breast_cancer.data, breast_cancer.target) print("Sizes of Train or Test Datasets : ", X_train.shape, X_test.shape, Y_train.shape, Y_test.shape) booster = lgb.LGBMModel(objective="binary", n_estimators=100, metric="auc") booster.fit(X_train, Y_train, eval_set=[(X_test, Y_test),], callbacks=[lgb.early_stopping(3)] ) from sklearn.metrics import accuracy_score test_preds = booster.predict(X_test) train_preds = booster.predict(X_train) test_preds = [1 if pred > 0.5 else 0 for pred in test_preds] train_preds = [1 if pred > 0.5 else 0 for pred in train_preds] print("\nAccuracy Score on Test Set : %.2f"%accuracy_score(Y_test, test_preds)) print("Accuracy Score on Train Set : %.2f"%accuracy_score(Y_train, train_preds))
输出
这将生成以下结果
Sizes of Train or Test Datasets : (426, 30) (143, 30) (426,) (143,) [1] valid_0's auc: 0.954328 Training until validation scores don't improve for 3 rounds [2] valid_0's auc: 0.959322 [3] valid_0's auc: 0.982938 [4] valid_0's auc: 0.988244 [5] valid_0's auc: 0.987203 [6] valid_0's auc: 0.98762 [7] valid_0's auc: 0.98814 Early stopping, best iteration is: [4] valid_0's auc: 0.988244 Accuracy Score on Test Set : 0.94 Accuracy Score on Train Set : 0.95
广告