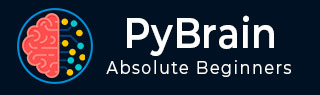
- PyBrain 教程
- PyBrain - 首页
- PyBrain - 概述
- PyBrain - 环境设置
- PyBrain - PyBrain 网络入门
- PyBrain - 使用网络
- PyBrain - 使用数据集
- PyBrain - 数据集类型
- PyBrain - 导入数据集数据
- PyBrain - 在网络上训练数据集
- PyBrain - 测试网络
- 使用前馈网络
- PyBrain - 使用循环网络
- 使用优化算法训练网络
- PyBrain - 层
- PyBrain - 连接
- PyBrain - 强化学习模块
- PyBrain - API 和工具
- PyBrain - 示例
- PyBrain 有用资源
- PyBrain - 快速指南
- PyBrain - 有用资源
- PyBrain - 讨论
PyBrain - 强化学习模块
强化学习 (RL) 是机器学习中的重要组成部分。强化学习使智能体能够根据环境的输入学习其行为。
强化学习过程中相互作用的组件如下:
- 环境
- 智能体
- 任务
- 实验
强化学习的布局如下:
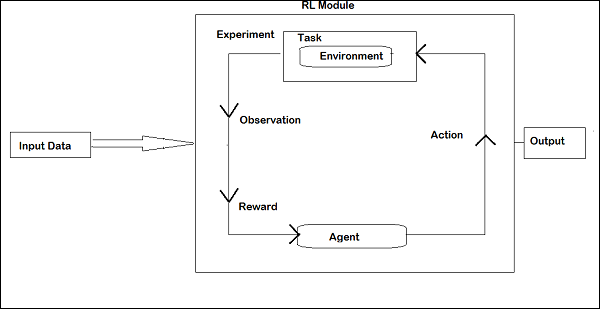
在 RL 中,智能体与环境迭代交互。在每次迭代中,智能体接收包含奖励的观察结果。然后,它选择动作并将其发送到环境。在每次迭代中,环境都会转移到新的状态,并且每次接收到的奖励都会被保存。
RL 智能体的目标是尽可能多地收集奖励。在迭代过程中,智能体的性能会与以良好方式运作的智能体的性能进行比较,并且性能差异会导致奖励或失败。RL 主要用于解决机器人控制、电梯、电信、游戏等问题。
让我们看看如何在 Pybrain 中使用 RL。
我们将使用迷宫环境,它将使用二维 NumPy 数组表示,其中 1 代表墙壁,0 代表空地。智能体的职责是在空地上移动并找到目标点。
以下是使用迷宫环境的分步流程。
步骤 1
使用以下代码导入所需的包:
from scipy import * import sys, time import matplotlib.pyplot as pylab # for visualization we are using mathplotlib from pybrain.rl.environments.mazes import Maze, MDPMazeTask from pybrain.rl.learners.valuebased import ActionValueTable from pybrain.rl.agents import LearningAgent from pybrain.rl.learners import Q, QLambda, SARSA #@UnusedImport from pybrain.rl.explorers import BoltzmannExplorer #@UnusedImport from pybrain.rl.experiments import Experiment from pybrain.rl.environments import Task
步骤 2
使用以下代码创建迷宫环境:
# create the maze with walls as 1 and 0 is a free field mazearray = array( [[1, 1, 1, 1, 1, 1, 1, 1, 1], [1, 0, 0, 1, 0, 0, 0, 0, 1], [1, 0, 0, 1, 0, 0, 1, 0, 1], [1, 0, 0, 1, 0, 0, 1, 0, 1], [1, 0, 0, 1, 0, 1, 1, 0, 1], [1, 0, 0, 0, 0, 0, 1, 0, 1], [1, 1, 1, 1, 1, 1, 1, 0, 1], [1, 0, 0, 0, 0, 0, 0, 0, 1], [1, 1, 1, 1, 1, 1, 1, 1, 1]] ) env = Maze(mazearray, (7, 7)) # create the environment, the first parameter is the maze array and second one is the goal field tuple
步骤 3
下一步是创建智能体。
智能体在 RL 中扮演着重要的角色。它将使用 getAction() 和 integrateObservation() 方法与迷宫环境进行交互。
智能体具有控制器(它将状态映射到动作)和学习器。
在 PyBrain 中,控制器就像一个模块,其输入是状态,并将它们转换为动作。
controller = ActionValueTable(81, 4) controller.initialize(1.)
ActionValueTable 需要 2 个输入,即状态和动作的数量。标准迷宫环境有 4 个动作:北、南、东、西。
现在我们将创建一个学习器。我们将使用 SARSA() 学习算法作为智能体使用的学习器。
learner = SARSA() agent = LearningAgent(controller, learner)
步骤 4
此步骤是将智能体添加到环境中。
为了将智能体连接到环境,我们需要一个名为任务的特殊组件。任务的作用是在环境中寻找目标,以及智能体如何通过动作获得奖励。
环境有其自己的任务。我们使用的迷宫环境具有 MDPMazeTask 任务。MDP 代表“马尔可夫决策过程”,这意味着智能体知道它在迷宫中的位置。环境将作为任务的参数。
task = MDPMazeTask(env)
步骤 5
将智能体添加到环境后的下一步是创建实验。
现在我们需要创建实验,以便任务和智能体可以相互协调。
experiment = Experiment(task, agent)
现在我们将运行实验 1000 次,如下所示:
for i in range(1000): experiment.doInteractions(100) agent.learn() agent.reset()
当以下代码执行时,环境将在智能体和任务之间运行 100 次。
experiment.doInteractions(100)
每次迭代后,它都会将新的状态返回给任务,任务决定应该将哪些信息和奖励传递给智能体。我们将在 for 循环内学习并重置智能体后绘制一个新表。
for i in range(1000): experiment.doInteractions(100) agent.learn() agent.reset() pylab.pcolor(table.params.reshape(81,4).max(1).reshape(9,9)) pylab.savefig("test.png")
这是完整的代码:
示例
maze.py
from scipy import * import sys, time import matplotlib.pyplot as pylab from pybrain.rl.environments.mazes import Maze, MDPMazeTask from pybrain.rl.learners.valuebased import ActionValueTable from pybrain.rl.agents import LearningAgent from pybrain.rl.learners import Q, QLambda, SARSA #@UnusedImport from pybrain.rl.explorers import BoltzmannExplorer #@UnusedImport from pybrain.rl.experiments import Experiment from pybrain.rl.environments import Task # create maze array mazearray = array( [[1, 1, 1, 1, 1, 1, 1, 1, 1], [1, 0, 0, 1, 0, 0, 0, 0, 1], [1, 0, 0, 1, 0, 0, 1, 0, 1], [1, 0, 0, 1, 0, 0, 1, 0, 1], [1, 0, 0, 1, 0, 1, 1, 0, 1], [1, 0, 0, 0, 0, 0, 1, 0, 1], [1, 1, 1, 1, 1, 1, 1, 0, 1], [1, 0, 0, 0, 0, 0, 0, 0, 1], [1, 1, 1, 1, 1, 1, 1, 1, 1]] ) env = Maze(mazearray, (7, 7)) # create task task = MDPMazeTask(env) #controller in PyBrain is like a module, for which the input is states and convert them into actions. controller = ActionValueTable(81, 4) controller.initialize(1.) # create agent with controller and learner - using SARSA() learner = SARSA() # create agent agent = LearningAgent(controller, learner) # create experiment experiment = Experiment(task, agent) # prepare plotting pylab.gray() pylab.ion() for i in range(1000): experiment.doInteractions(100) agent.learn() agent.reset() pylab.pcolor(controller.params.reshape(81,4).max(1).reshape(9,9)) pylab.savefig("test.png")
输出
python maze.py
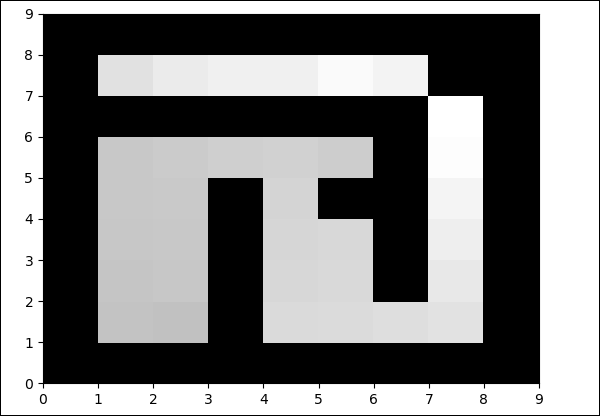
空地中的颜色将在每次迭代时更改。