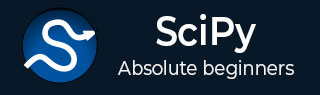
- SciPy 教程
- SciPy - 首页
- SciPy - 简介
- SciPy - 环境设置
- SciPy - 基本功能
- SciPy - 聚类
- SciPy - 常量
- SciPy - FFTpack
- SciPy - 积分
- SciPy - 插值
- SciPy - 输入和输出
- SciPy - 线性代数 (Linalg)
- SciPy - N维图像处理 (Ndimage)
- SciPy - 优化
- SciPy - 统计
- SciPy - 压缩稀疏图 (CSGraph)
- SciPy - 空间
- SciPy - 正交距离回归 (ODR)
- SciPy - 特殊函数包
- SciPy 有用资源
- SciPy - 参考
- SciPy - 快速指南
- SciPy - 有用资源
- SciPy - 讨论
SciPy - integrate.simpson() 方法
SciPy 的 integrate.simpson() 方法用于使用辛普森法则逼近函数的积分。此法则适用于偶数个区间。
假设有两个样本,N(偶数)和 N-1(奇数)。为了处理奇数区间的这种情况,simpson() 方法提供了一个 even 参数来控制它。
语法
以下是 SciPy integrate.simpson() 方法的语法:
simpson(y, x)
参数
此方法接受两个参数:
- y:此参数表示简单的数学运算,例如 sin()、exp() 等。
- x:使用此参数,我们可以表示以下内容:数组和内置函数。
返回值
此方法返回浮点值作为结果。
示例 1
以下是一个基本示例,演示了 SciPy integrate.simpson() 方法的使用。
import numpy as np from scipy import integrate # 100 sample points between 0 and 10 x = np.linspace(0, 10, 100) y = x**2 res_integral = integrate.simpson(y, x) print("The resultant value is ", res_integral)
输出
以上代码产生以下结果:
The resultant value is 333.333505101692
示例 2
在这里,我们使用辛普森法则和 50 个采样点,对从 0 到 pi 区间的 sin(x) 函数进行积分。
import numpy as np from scipy.integrate import simpson # 50 sample points between 0 and π x = np.linspace(0, np.pi, 50) y = np.sin(x) res_integral = simpson(y, x) print("The resultant value is ", res_integral)
输出
以上代码产生以下结果:
The resultant value is 1.999999483788026
说明:这是一个近似值,非常接近 2,它在处理奇数区间时确定了高精度。
示例 3
下面是另一个使用辛普森法则的程序,它将给定的输入显示为列表数组 (x)。然后它使用 exp(),这有助于设置少量数据点(偶数)。最后,使用辛普森方法获得结果(近似值)。
import numpy as np from scipy.integrate import simpson x = np.array([0, 0.1, 0.4, 0.8, 1.0]) y = np.exp(x) res_integral = simpson(y, x) print("The resultant value is ", res_integral)
输出
以上代码产生以下结果:
The resultant value is 1.7173084152992835
scipy_reference.htm
广告