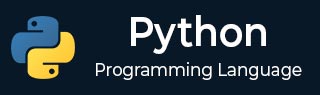
- Python 基础
- Python - 首页
- Python - 概述
- Python - 历史
- Python - 特性
- Python vs C++
- Python - Hello World 程序
- Python - 应用领域
- Python - 解释器
- Python - 环境搭建
- Python - 虚拟环境
- Python - 基本语法
- Python - 变量
- Python - 数据类型
- Python - 类型转换
- Python - Unicode 系统
- Python - 字面量
- Python - 运算符
- Python - 算术运算符
- Python - 比较运算符
- Python - 赋值运算符
- Python - 逻辑运算符
- Python - 位运算符
- Python - 成员运算符
- Python - 身份运算符
- Python - 运算符优先级
- Python - 注释
- Python - 用户输入
- Python - 数字
- Python - 布尔值
- Python 控制语句
- Python - 控制流
- Python - 决策
- Python - if 语句
- Python - if else
- Python - 嵌套 if
- Python - match-case 语句
- Python - 循环
- Python - for 循环
- Python - for-else 循环
- Python - while 循环
- Python - break 语句
- Python - continue 语句
- Python - pass 语句
- Python - 嵌套循环
- Python 函数与模块
- Python - 函数
- Python - 默认参数
- Python - 关键字参数
- Python - 仅限关键字参数
- Python - 位置参数
- Python - 仅限位置参数
- Python - 可变参数
- Python - 变量作用域
- Python - 函数注解
- Python - 模块
- Python - 内置函数
- Python 字符串
- Python - 字符串
- Python - 字符串切片
- Python - 修改字符串
- Python - 字符串连接
- Python - 字符串格式化
- Python - 转义字符
- Python - 字符串方法
- Python - 字符串练习
- Python 列表
- Python - 列表
- Python - 访问列表元素
- Python - 修改列表元素
- Python - 添加列表元素
- Python - 删除列表元素
- Python - 遍历列表
- Python - 列表推导式
- Python - 排序列表
- Python - 复制列表
- Python - 合并列表
- Python - 列表方法
- Python - 列表练习
- Python 元组
- Python - 元组
- Python - 访问元组元素
- Python - 更新元组
- Python - 解包元组
- Python - 遍历元组
- Python - 合并元组
- Python - 元组方法
- Python - 元组练习
- Python 集合
- Python - 集合
- Python - 访问集合元素
- Python - 添加集合元素
- Python - 删除集合元素
- Python - 遍历集合
- Python - 合并集合
- Python - 复制集合
- Python - 集合运算符
- Python - 集合方法
- Python - 集合练习
- Python 字典
- Python - 字典
- Python - 访问字典元素
- Python - 修改字典元素
- Python - 添加字典元素
- Python - 删除字典元素
- Python - 字典视图对象
- Python - 遍历字典
- Python - 复制字典
- Python - 嵌套字典
- Python - 字典方法
- Python - 字典练习
- Python 数组
- Python - 数组
- Python - 访问数组元素
- Python - 添加数组元素
- Python - 删除数组元素
- Python - 遍历数组
- Python - 复制数组
- Python - 反转数组
- Python - 排序数组
- Python - 合并数组
- Python - 数组方法
- Python - 数组练习
- Python 文件处理
- Python - 文件处理
- Python - 写入文件
- Python - 读取文件
- Python - 重命名和删除文件
- Python - 目录
- Python - 文件方法
- Python - OS 文件/目录方法
- Python - OS 路径方法
- 面向对象编程
- Python - OOP 概念
- Python - 类与对象
- Python - 类属性
- Python - 类方法
- Python - 静态方法
- Python - 构造函数
- Python - 访问修饰符
- Python - 继承
- Python - 多态
- Python - 方法重写
- Python - 方法重载
- Python - 动态绑定
- Python - 动态类型
- Python - 抽象
- Python - 封装
- Python - 接口
- Python - 包
- Python - 内部类
- Python - 匿名类和对象
- Python - 单例类
- Python - 包装类
- Python - 枚举
- Python - 反射
- Python 错误与异常
- Python - 语法错误
- Python - 异常
- Python - try-except 块
- Python - try-finally 块
- Python - 抛出异常
- Python - 异常链
- Python - 嵌套 try 块
- Python - 用户自定义异常
- Python - 日志记录
- Python - 断言
- Python - 内置异常
- Python 多线程
- Python - 多线程
- Python - 线程生命周期
- Python - 创建线程
- Python - 启动线程
- Python - 加入线程
- Python - 线程命名
- Python - 线程调度
- Python - 线程池
- Python - 主线程
- Python - 线程优先级
- Python - 守护线程
- Python - 线程同步
- Python 同步
- Python - 线程间通信
- Python - 线程死锁
- Python - 中断线程
- Python 网络编程
- Python - 网络编程
- Python - Socket 编程
- Python - URL 处理
- Python - 泛型
- Python 库
- NumPy 教程
- Pandas 教程
- SciPy 教程
- Matplotlib 教程
- Django 教程
- OpenCV 教程
- Python 杂项
- Python - 日期与时间
- Python - 数学
- Python - 迭代器
- Python - 生成器
- Python - 闭包
- Python - 装饰器
- Python - 递归
- Python - 正则表达式
- Python - PIP
- Python - 数据库访问
- Python - 弱引用
- Python - 序列化
- Python - 模板
- Python - 输出格式化
- Python - 性能测量
- Python - 数据压缩
- Python - CGI 编程
- Python - XML 处理
- Python - GUI 编程
- Python - 命令行参数
- Python - 文档字符串
- Python - JSON
- Python - 发送邮件
- Python - 扩展
- Python - 工具/实用程序
- Python - GUI
- Python 高级概念
- Python - 抽象基类
- Python - 自定义异常
- Python - 高阶函数
- Python - 对象内部
- Python - 内存管理
- Python - 元类
- Python - 使用元类进行元编程
- Python - 模拟和存根
- Python - Monkey Patching
- Python - 信号处理
- Python - 类型提示
- Python - 自动化教程
- Python - Humanize 包
- Python - 上下文管理器
- Python - 协程
- Python - 描述符
- Python - 诊断和修复内存泄漏
- Python - 不可变数据结构
- Python 有用资源
- Python - 问答
- Python - 在线测验
- Python - 快速指南
- Python - 参考
- Python - 速查表
- Python - 项目
- Python - 有用资源
- Python - 讨论
- Python 编译器
- NumPy 编译器
- Matplotlib 编译器
- SciPy 编译器
Python statistics.linear_regression() 函数
Python 的statistics.linear_regression()函数使用最小二乘法返回简单线性参数的斜率和截距。
简单线性回归描述了自变量x和因变量y之间的关系,用线性函数表示,即:
y = 斜率*x + 截距 + 噪声
这里,斜率和截距是估计的回归参数,噪声表示线性回归中给定数据的可变性。这些值等于变量的预测值和实际值之间的差异。
输入变量必须长度相同,并且自变量x不能是常数;否则会抛出StatisticsError异常。
语法
以下是statistics.linear_regression()函数的基本语法。
statistics.linear_regression(x, y, /, *, proportional = False)
参数
此函数采用x和y值,其中x是自变量,y是因变量。
返回值
回归函数返回斜率和截距。
示例 1
这里,我们演示了statistics.linear_regression()函数来确定x和y值。
import statistics x = [0, 1, 2, 3, 4, 5, 6, 7] y = [12, 13, 12, 15, 16, 17, 18, 19] slope, intercept = statistics.linear_regression(x, y) print("slope - ", slope) print("Intercept - ", intercept)
输出
结果如下所示:
slope - 1.0714285714285714 Intercept - 11.5
示例 2
在下面的示例中,我们使用statistics.linear_regression()函数计算负数。
import statistics x = [-3, -4, -5, -7, -9, -4, -2] y = [-13, -31, -14, -56, -35, -43, -23] slope, intercept = statistics.linear_regression(x, y) print("slope - ", slope) print("Intercept -", intercept)
输出
我们将得到如下输出:
slope - 3.262295081967214 Intercept - -14.868852459016392
示例 3
在下面的例子中,我们假设如果蒙提·派森保持和平,预测到2019年为止制作的蒙提·派森电影的累计数量。
import statistics x = [1971, 1975, 1979, 1982, 1983] y = [1, 2, 3, 4, 5] slope, intercept = statistics.linear_regression(x, y) print(round(slope * 2019 + intercept))
输出
这将产生以下结果:
16
示例 4
这里,我们计算给定值的线性回归(假设x = 100)。
import statistics x = [19, 75, 97, 82, 31] y = [21, 12, 43, 74, 35] slope, intercept = statistics.linear_regression(x, y) print(round(slope * 100 + intercept))
输出
输出如下所示:
49
python_modules.htm
广告