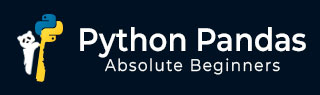
- Python Pandas 教程
- Python Pandas - 首页
- Python Pandas - 简介
- Python Pandas - 环境设置
- Python Pandas - 基础
- Python Pandas - 数据结构介绍
- Python Pandas - 索引对象
- Python Pandas - Panel
- Python Pandas - 基本功能
- Python Pandas - 索引和数据选择
- Python Pandas - Series
- Python Pandas - Series
- Python Pandas - 切片 Series 对象
- Python Pandas - Series 对象的属性
- Python Pandas - Series 对象的算术运算
- Python Pandas - 将 Series 转换为其他对象
- Python Pandas - DataFrame
- Python Pandas - DataFrame
- Python Pandas - 访问 DataFrame
- Python Pandas - 切片 DataFrame 对象
- Python Pandas - 修改 DataFrame
- Python Pandas - 从 DataFrame 中删除行
- Python Pandas - DataFrame 的算术运算
- Python Pandas - I/O 工具
- Python Pandas - I/O 工具
- Python Pandas - 使用 CSV 格式
- Python Pandas - 读取和写入 JSON 文件
- Python Pandas - 从 Excel 文件读取数据
- Python Pandas - 将数据写入 Excel 文件
- Python Pandas - 使用 HTML 数据
- Python Pandas - 剪贴板
- Python Pandas - 使用 HDF5 格式
- Python Pandas - 与 SQL 的比较
- Python Pandas - 数据处理
- Python Pandas - 排序
- Python Pandas - 重索引
- Python Pandas - 迭代
- Python Pandas - 连接
- Python Pandas - 统计函数
- Python Pandas - 描述性统计
- Python Pandas - 处理文本数据
- Python Pandas - 函数应用
- Python Pandas - 选项和自定义
- Python Pandas - 窗口函数
- Python Pandas - 聚合
- Python Pandas - 合并/连接
- Python Pandas - 多层索引
- Python Pandas - 多层索引基础
- Python Pandas - 使用多层索引进行索引
- Python Pandas - 使用多层索引进行高级重索引
- Python Pandas - 重命名多层索引标签
- Python Pandas - 对多层索引进行排序
- Python Pandas - 二元运算
- Python Pandas - 二元比较运算
- Python Pandas - 布尔索引
- Python Pandas - 布尔掩码
- Python Pandas - 数据重塑和透视
- Python Pandas - 透视
- Python Pandas - 堆叠和取消堆叠
- Python Pandas - 熔化
- Python Pandas - 计算虚拟变量
- Python Pandas - 分类数据
- Python Pandas - 分类数据
- Python Pandas - 分类数据的排序
- Python Pandas - 比较分类数据
- Python Pandas - 处理缺失数据
- Python Pandas - 缺失数据
- Python Pandas - 填充缺失数据
- Python Pandas - 缺失值的插值
- Python Pandas - 删除缺失数据
- Python Pandas - 处理缺失数据
- Python Pandas - 处理重复项
- Python Pandas - 重复数据
- Python Pandas - 计数和检索唯一元素
- Python Pandas - 重复标签
- Python Pandas - 分组和聚合
- Python Pandas - GroupBy
- Python Pandas - 时间序列数据
- Python Pandas - 日期功能
- Python Pandas - 时间增量
- Python Pandas - 稀疏数据结构
- Python Pandas - 稀疏数据
- Python Pandas - 可视化
- Python Pandas - 可视化
- Python Pandas - 其他概念
- Python Pandas - 注意事项
- Python Pandas 资源
- Python Pandas - 快速指南
- Python Pandas - 资源
- Python Pandas - 讨论
Python Pandas - 处理缺失数据
在处理数据时,您经常会遇到缺失值,在 Pandas 中表示为 NaN(非数字)。处理缺失值需要更多注意,因为 NaN 值会传播到大多数算术运算中,这可能会改变结果。
Pandas 提供了灵活的方法来管理计算期间的缺失数据,允许您控制这些值如何影响您的结果。在本教程中,我们将学习 Pandas 如何在计算期间处理缺失数据,包括算术运算、描述性统计和累积运算。
带有缺失数据的算术运算
在 Pandas 对象之间执行算术运算时,缺失值 (NaN) 默认情况下会传播。例如,当您将两个包含 NaN 值的 Series 相加时,结果中也会在任何一个 Series 中存在缺失值的地方出现 NaN。
示例
以下示例演示了对包含缺失值的两个 Series 对象执行算术运算。
import pandas as pd import numpy as np # Create 2 input series objects ser1 = pd.Series([1, np.nan, np.nan, 2]) ser2 = pd.Series([2, np.nan, 1, np.nan]) # Display the series print("Input Series 1:\n",ser1) print("\nInput Series 2:\n",ser2) # Adding two series with NaN values result = ser1 + ser2 print('\nResult After adding Two series:\n',result)
以下是上述代码的输出:
Input Series 1: 0 1.0 1 NaN 2 NaN 3 2.0 dtype: float64 Input Series 2: 0 2.0 1 NaN 2 1.0 3 NaN dtype: float64 Result After adding Two series: 0 3.0 1 NaN 2 NaN 3 NaN dtype: float64
处理描述性统计中的缺失数据
Pandas 库提供了多种计算描述性统计的方法,例如求和、计算乘积或查找累积和或乘积。这些方法旨在高效地处理缺失数据。
示例:带有缺失值的求和
在对包含缺失值的数据求和时,会排除 NaN 值。这允许您即使在某些数据缺失的情况下也能计算有意义的总计。
以下示例使用sum()函数对 DataFrame 列执行求和运算。默认情况下,求和运算中会跳过 NaN 值。
import pandas as pd import numpy as np # Create a sample DataFrame data = {'A': [np.nan, 2, np.nan, 4], 'B': [5, 6, 7, 8]} df = pd.DataFrame(data) # Display the input DataFrame print("Input DataFrame:\n", df) # Summing a column with NaN values result = df['A'].sum() print('\nResult After Summing the values of a column:\n',result)
以下是上述代码的输出:
Input DataFrame:
A | B | |
---|---|---|
0 | NaN | 5 |
1 | 2.0 | 6 |
2 | NaN | 7 |
3 | 4.0 | 8 |
示例:带有缺失值的乘积计算
与求和类似,当计算包含缺失数据 (NaN) 的值的乘积时,将其视为 1。这确保缺失值不会改变最终乘积。
以下示例使用 pandas df.prod() 函数计算 pandas 对象的乘积。
import pandas as pd import numpy as np # Create a sample DataFrame data = {'A': [np.nan, 2, np.nan, 4], 'B': [5, 6, np.nan, np.nan]} df = pd.DataFrame(data) # Display the input DataFrame print("Input DataFrame:\n", df) # Product with NaN values result = df.prod() print('\nResult After Product the values of a DataFrame:\n',result)
以下是上述代码的输出:
Input DataFrame:
A | B | |
---|---|---|
0 | NaN | 5.0 |
1 | 2.0 | 6.0 |
2 | NaN | NaN |
3 | 4.0 | NaN |
带有缺失数据的累积运算
Pandas 提供了诸如cumsum()和cumprod()之类的累积方法来生成运行总计或乘积。默认情况下,这些方法会忽略缺失值,但在输出中保留它们。如果要将缺失数据包含在计算中,可以将skipna参数设置为 False。
示例:带有缺失值的累积和
以下示例演示了如何使用df.cumsum()方法计算包含缺失值的 DataFrame 的累积和。
import pandas as pd import numpy as np # Create a sample DataFrame data = {'A': [np.nan, 2, np.nan, 4], 'B': [5, 6, np.nan, np.nan]} df = pd.DataFrame(data) # Display the input DataFrame print("Input DataFrame:\n", df) # Calculate cumulative sum by ignoring NaN print('Cumulative sum by ignoring NaN:\n',df.cumsum())
以下是上述代码的输出:
Input DataFrame:
A | B | |
---|---|---|
0 | NaN | 5.0 |
1 | 2.0 | 6.0 |
2 | NaN | NaN |
3 | 4.0 | NaN |
A | B | |
---|---|---|
0 | NaN | 5.0 |
1 | 2.0 | 11.0 |
2 | NaN | NaN |
3 | 6.0 | NaN |
从上述输出可以看出,跳过了缺失值,并为可用值计算了累积和。
示例:在累积和中包含 NaN
此示例演示了如何通过将skipna=False设置在df.cumsum()方法中来包含缺失值,从而执行累积和。
import pandas as pd import numpy as np # Create a sample DataFrame data = {'A': [np.nan, 2, np.nan, 4], 'B': [5, 6, np.nan, np.nan]} df = pd.DataFrame(data) # Display the input DataFrame print("Input DataFrame:\n", df) # Calculate the cumulative sum by preserving NaN print('Cumulative sum by including NaN:\n', df.cumsum(skipna=False))
以下是上述代码的输出:
Input DataFrame:
A | B | |
---|---|---|
0 | NaN | 5.0 |
1 | 2.0 | 6.0 |
2 | NaN | NaN |
3 | 4.0 | NaN |
A | B | |
---|---|---|
0 | NaN | 5.0 |
1 | NaN | 11.0 |
2 | NaN | NaN |
3 | NaN | NaN |
使用skipna=False,累积和会在遇到 NaN 值时停止,并且所有后续值也变为 NaN。