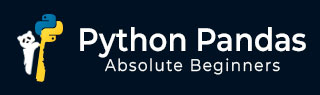
- Python Pandas 教程
- Python Pandas - 首页
- Python Pandas - 简介
- Python Pandas - 环境设置
- Python Pandas - 基础知识
- Python Pandas - 数据结构介绍
- Python Pandas - 索引对象
- Python Pandas - 面板(Panel)
- Python Pandas - 基本功能
- Python Pandas - 索引和数据选择
- Python Pandas - Series
- Python Pandas - Series
- Python Pandas - Series 对象切片
- Python Pandas - Series 对象的属性
- Python Pandas - Series 对象的算术运算
- Python Pandas - 将 Series 转换为其他对象
- Python Pandas - DataFrame
- Python Pandas - DataFrame
- Python Pandas - 访问 DataFrame
- Python Pandas - DataFrame 对象切片
- Python Pandas - 修改 DataFrame
- Python Pandas - 从 DataFrame 中删除行
- Python Pandas - DataFrame 的算术运算
- Python Pandas - I/O 工具
- Python Pandas - I/O 工具
- Python Pandas - 使用 CSV 格式
- Python Pandas - 读取和写入 JSON 文件
- Python Pandas - 从 Excel 文件读取数据
- Python Pandas - 将数据写入 Excel 文件
- Python Pandas - 使用 HTML 数据
- Python Pandas - 剪贴板
- Python Pandas - 使用 HDF5 格式
- Python Pandas - 与 SQL 的比较
- Python Pandas - 数据处理
- Python Pandas - 排序
- Python Pandas - 重新索引
- Python Pandas - 迭代
- Python Pandas - 级联
- Python Pandas - 统计函数
- Python Pandas - 描述性统计
- Python Pandas - 使用文本数据
- Python Pandas - 函数应用
- Python Pandas - 选项和自定义
- Python Pandas - 窗口函数
- Python Pandas - 聚合
- Python Pandas - 合并/连接
- Python Pandas - 多索引
- Python Pandas - 多索引基础
- Python Pandas - 使用多索引进行索引
- Python Pandas - 使用多索引的高级重新索引
- Python Pandas - 重命名多索引标签
- Python Pandas - 对多索引排序
- Python Pandas - 二元运算
- Python Pandas - 二元比较运算
- Python Pandas - 布尔索引
- Python Pandas - 布尔掩码
- Python Pandas - 数据重塑和透视
- Python Pandas - 透视表
- Python Pandas - 堆叠和取消堆叠
- Python Pandas - 熔化(Melting)
- Python Pandas - 计算虚拟变量
- Python Pandas - 分类数据
- Python Pandas - 分类数据
- Python Pandas - 分类数据的排序
- Python Pandas - 分类数据的比较
- Python Pandas - 处理缺失数据
- Python Pandas - 缺失数据
- Python Pandas - 填充缺失数据
- Python Pandas - 缺失值的插值
- Python Pandas - 删除缺失数据
- Python Pandas - 使用缺失数据的计算
- Python Pandas - 处理重复项
- Python Pandas - 重复数据
- Python Pandas - 计数和检索唯一元素
- Python Pandas - 重复标签
- Python Pandas - 分组和聚合
- Python Pandas - GroupBy
- Python Pandas - 时间序列数据
- Python Pandas - 日期功能
- Python Pandas - Timedelta
- Python Pandas - 稀疏数据结构
- Python Pandas - 稀疏数据
- Python Pandas - 可视化
- Python Pandas - 可视化
- Python Pandas - 附加概念
- Python Pandas - 警告和陷阱
- Python Pandas 有用资源
- Python Pandas - 快速指南
- Python Pandas - 有用资源
- Python Pandas - 讨论
Python Pandas - 计算虚拟变量
虚拟变量,也称为指示变量,是表示分类数据的二元 (0 或 1) 变量。在数据分析中,尤其是在处理分类数据时,通常需要将分类变量转换为数值格式。一种常用的方法是使用虚拟变量。
本教程将介绍如何在 Python Pandas 中使用 get_dummies() 和 from_dummies() 函数创建虚拟变量。
使用 get_dummies() 创建虚拟变量
Pandas 中的 get_dummies() 函数用于将 Series 或 DataFrame 的分类变量转换为虚拟变量。
示例:创建虚拟变量的基本示例
这是一个使用 pandas.get_dummies() 函数创建虚拟变量的基本示例。
import pandas as pd import numpy as np # Create a DataFrame df = pd.DataFrame({"keys": list("aeeioou"), "values": range(7)}) # Display the Input DataFrame print('Input DataFrame:\n',df) # Create dummy variables for the keys column dummies = pd.get_dummies(df["keys"]) print('Resultant Dummy Variables:\n',dummies)
以下是上述代码的输出:
Input DataFrame:
keys | values |
---|---|
a | 0 |
e | 1 |
e | 2 |
i | 3 |
o | 4 |
o | 5 |
u | 6 |
a | e | i | o | u | |
---|---|---|---|---|---|
0 | True | False | False | False | False |
1 | False | True | False | False | False |
2 | False | True | False | False | False |
3 | False | False | True | False | False |
4 | False | False | False | True | False |
5 | False | False | False | True | False |
6 | False | False | False | False | True |
使用前缀创建虚拟变量
get_dummies() 函数允许您通过使用 prefix 参数,在将 Pandas 对象的分类变量转换为虚拟变量时,为虚拟变量列名添加前缀。
示例
此示例演示了使用 pandas.get_dummies() 函数使用前缀创建虚拟变量。
import pandas as pd import numpy as np # Create a DataFrame df = pd.DataFrame({"keys": list("aeeioou"), "values": range(7)}) # Display the Input DataFrame print('Input DataFrame:\n',df) # Create dummy variables for the keys column dummies = pd.get_dummies(df["keys"], prefix="Col_") print('Resultant Dummy Variables with Prefix:\n',dummies)
以下是上述代码的输出:
Input DataFrame:
keys | values |
---|---|
a | 0 |
e | 1 |
e | 2 |
i | 3 |
o | 4 |
o | 5 |
u | 6 |
Col__a | Col__e | Col__i | Col__o | Col__u | |
---|---|---|---|---|---|
0 | True | False | False | False | False |
1 | False | True | False | False | False |
2 | False | True | False | False | False |
3 | False | False | True | False | False |
4 | False | False | False | True | False |
5 | False | False | False | True | False |
6 | False | False | False | False | True |
创建虚拟变量时处理共线性
为了避免统计模型中的共线性问题,您可以通过将 drop_first 参数设置为 True 来删除第一个虚拟变量。
示例
此示例使用 pandas.get_dummies() 函数的 drop_first 参数删除第一个虚拟变量。
import pandas as pd import numpy as np # Create a DataFrame df = pd.DataFrame({"keys": list("aeeioou"), "values": range(7)}) # Display the Input DataFrame print('Input DataFrame:\n',df) # Create dummy variables for the keys column dummies = pd.get_dummies(df["keys"], drop_first=True) print('Resultant Dummy Variables with Prefix:\n',dummies)
以下是上述代码的输出:
Input DataFrame:
keys | values |
---|---|
a | 0 |
e | 1 |
e | 2 |
i | 3 |
o | 4 |
o | 5 |
u | 6 |
e | i | o | u | |
---|---|---|---|---|
0 | False | False | False | False |
1 | True | False | False | False |
2 | True | False | False | False |
3 | False | True | False | False |
4 | False | False | True | False |
5 | False | False | True | False |
6 | False | False | False | True |
从虚拟变量创建分类变量
pandas.from_dummies() 函数用于将 get_dummies() 的输出转换回分类 Series。
示例
此示例演示了使用 pandas.from_dummies() 函数从虚拟变量创建分类 Series。
import pandas as pd import numpy as np # Create a DataFrame with dummy variables df = pd.DataFrame({"Col_a": [0, 1, 0], "Col_b": [1, 0, 1]}) # Display the Input DataFrame print('Input DataFrame:\n',df) # Convert the Dummies back to categorical original_series = pd.from_dummies(df, sep="_") print('Resultant Categorical Variables:\n',original_series )
以下是上述代码的输出:
Input DataFrame:
Col_a | Col_b |
---|---|
0 | 1 |
1 | 0 |
0 | 1 |
Col |
---|
b |
a |
b |
广告