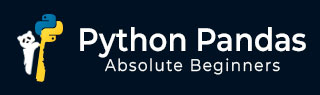
- Python Pandas 教程
- Python Pandas - 首页
- Python Pandas - 简介
- Python Pandas - 环境设置
- Python Pandas - 基础知识
- Python Pandas - 数据结构介绍
- Python Pandas - 索引对象
- Python Pandas - Panel
- Python Pandas - 基本功能
- Python Pandas - 索引和数据选择
- Python Pandas - Series
- Python Pandas - Series
- Python Pandas - Series 对象切片
- Python Pandas - Series 对象的属性
- Python Pandas - Series 对象的算术运算
- Python Pandas - Series 到其他对象的转换
- Python Pandas - DataFrame
- Python Pandas - DataFrame
- Python Pandas - 访问 DataFrame
- Python Pandas - DataFrame 切片
- Python Pandas - 修改 DataFrame
- Python Pandas - 从 DataFrame 中删除行
- Python Pandas - DataFrame 的算术运算
- Python Pandas - I/O 工具
- Python Pandas - I/O 工具
- Python Pandas - 使用 CSV 格式
- Python Pandas - 读取和写入 JSON 文件
- Python Pandas - 从 Excel 文件读取数据
- Python Pandas - 将数据写入 Excel 文件
- Python Pandas - 使用 HTML 数据
- Python Pandas - 剪贴板
- Python Pandas - 使用 HDF5 格式
- Python Pandas - 与 SQL 的比较
- Python Pandas - 数据处理
- Python Pandas - 排序
- Python Pandas - 重新索引
- Python Pandas - 迭代
- Python Pandas - 连接
- Python Pandas - 统计函数
- Python Pandas - 描述性统计
- Python Pandas - 使用文本数据
- Python Pandas - 函数应用
- Python Pandas - 选项和自定义
- Python Pandas - 窗口函数
- Python Pandas - 聚合
- Python Pandas - 合并/连接
- Python Pandas - 多级索引
- Python Pandas - 多级索引基础
- Python Pandas - 使用多级索引进行索引
- Python Pandas - 使用多级索引的高级重新索引
- Python Pandas - 重命名多级索引标签
- Python Pandas - 对多级索引排序
- Python Pandas - 二元运算
- Python Pandas - 二元比较运算
- Python Pandas - 布尔索引
- Python Pandas - 布尔掩码
- Python Pandas - 数据重塑和透视
- Python Pandas - 透视表
- Python Pandas - 堆叠和取消堆叠
- Python Pandas - 熔化
- Python Pandas - 计算虚拟变量
- Python Pandas - 分类数据
- Python Pandas - 分类数据
- Python Pandas - 分类数据的排序和排序
- Python Pandas - 比较分类数据
- Python Pandas - 处理缺失数据
- Python Pandas - 缺失数据
- Python Pandas - 填充缺失数据
- Python Pandas - 缺失值的插值
- Python Pandas - 删除缺失数据
- Python Pandas - 使用缺失数据进行计算
- Python Pandas - 处理重复项
- Python Pandas - 重复数据
- Python Pandas - 计数和检索唯一元素
- Python Pandas - 重复标签
- Python Pandas - 分组和聚合
- Python Pandas - GroupBy
- Python Pandas - 时间序列数据
- Python Pandas - 日期功能
- Python Pandas - Timedelta
- Python Pandas - 稀疏数据结构
- Python Pandas - 稀疏数据
- Python Pandas - 可视化
- Python Pandas - 可视化
- Python Pandas - 其他概念
- Python Pandas - 警告和注意事项
- Python Pandas 有用资源
- Python Pandas - 快速指南
- Python Pandas - 有用资源
- Python Pandas - 讨论
Python Pandas - DataFrame 切片
Pandas DataFrame 切片是根据位置和标签提取特定行、列或数据子集的过程。在处理大型数据集时,DataFrame 切片是一种常见的操作,它类似于 Python 列表和 NumPy ndarrays,DataFrame 切片使用 [] 运算符和特定的切片属性,如 .iloc[] 和 .loc[] 来有效地检索数据。
在本教程中,我们将学习如何使用基于位置和基于标签的索引来切片 Pandas DataFrame。
Pandas DataFrame 切片的介绍
Pandas DataFrame 切片使用两个主要属性执行,它们是:
.iloc[]:基于位置(基于整数的索引)进行切片。
.loc[]:基于标签(索引标签或列标签)进行切片。
让我们学习所有可能的 Pandas DataFrame 切片方法。
按位置切片 DataFrame
Pandas DataFrame.iloc[] 属性用于基于 DataFrame 行和列的整数位置(即基于整数的索引)来切片 DataFrame。
以下是使用 .iloc[] 属性切片 DataFrame 的语法:
DataFrame.iloc[row_start:row_end, column_start:column_end]
其中,row_start 和 row_end 表示 DataFrame 行的起始和结束整数索引值。类似地,column_start 和 column_end 是列索引值。
示例:按位置切片 DataFrame 行
以下示例演示如何使用 DataFrame.iloc[] 属性切片 DataFrame 行。
import pandas as pd # Create a Pandas DataFrame df = pd.DataFrame([['a','b'], ['c','d'], ['e','f'], ['g','h']], columns=['col1', 'col2']) # Display the DataFrame print("Input DataFrame:") print(df) # Slice rows based on position result = df.iloc[1:3, :] print("Output:") print(result)
以上代码的输出如下:
Input DataFrame: col1 col2 0 a b 1 c d 2 e f 3 g h Output: col1 col2 1 c d 2 e f
按标签切片 DataFrame
Pandas DataFrame.loc[] 属性用于基于行和列的标签来切片 DataFrame。
以下是使用 .loc[] 属性切片 DataFrame 的语法:
DataFrame.loc[row_label_start:row_label_end, column_label_start:column_label_end]
其中,row_label_start 和 row_label_end 表示 DataFrame 行的起始和结束标签。类似地,column_label_start 和 column_label_end 是列标签。
示例:使用 .loc[] 切片 DataFrame 行和列
以下示例演示如何使用 .loc[] 属性通过其标签来切片 DataFrame 行和列。
import pandas as pd # Create a DataFrame with labeled indices df = pd.DataFrame([['a','b'], ['c','d'], ['e','f'], ['g','h']], columns=['col1', 'col2'], index=['r1', 'r2', 'r3', 'r4']) # Display the DataFrame print("Original DataFrame:") print(df) # Slice rows and columns by label result = df.loc['r1':'r3', 'col1'] print("Output:") print(result)
以上代码的输出如下:
Original DataFrame: col1 col2 r1 a b r2 c d r3 e f r4 g h Output: r1 a r2 c r3 e Name: col1, dtype: object
DataFrame 列切片
与上述行切片类似,Pandas DataFrame 列切片也可以使用 .iloc[] 进行位置切片,使用 .loc[] 进行标签切片。
示例:使用 iloc[] 进行列切片
以下示例根据其整数位置切片 DataFrame 列。
import pandas as pd data = {'A': [1, 2, 3], 'B': [4, 5, 6], 'C': [7, 8, 9]} df = pd.DataFrame(data) # Slice a single column col_A = df.iloc[:, 0] print("Slicing a single column A using iloc[]:") print(col_A) # Slice multiple columns cols_AB = df.iloc[:, 0:2] print("Slicing multiple columns A and B using iloc[]:") print(cols_AB)
以上代码的输出如下:
Slicing a single column A using iloc[]: 0 1 1 2 2 3 Name: A, dtype: int64 Slicing multiple columns A and B using iloc[]: A B 0 1 4 1 2 5 2 3 6
示例:使用 loc[] 进行列切片
此示例使用 .loc[] 属性按其标签切片 DataFrame 列。
import pandas as pd data = {'A': [1, 2, 3], 'B': [4, 5, 6], 'C': [7, 8, 9]} df = pd.DataFrame(data) # Slice a single column by label col_A = df.loc[:, 'A'] print("Slicing a single column A using loc[]:") print(col_A) # Slice multiple columns by label cols_AB = df.loc[:, 'A':'B'] print("Slicing Multiple columns A and B using loc[]:") print(cols_AB)
以上代码的输出如下:
Slicing a single column A using loc[]: 0 1 1 2 2 3 Name: A, dtype: int64 Slicing Multiple columns A and B using loc[]: A B 0 1 4 1 2 5 2 3 6
切片后修改值
切片 DataFrame 后,可以直接修改切片的值。这可以通过为所选元素赋值新值来完成。
示例
此示例演示如何直接修改切片后的 DataFrame 值。
import pandas as pd # Create a DataFrame df = pd.DataFrame([['a', 'b'], ['c', 'd'], ['e', 'f'], ['g', 'h']], columns=['col1', 'col2']) # Display the Original DataFrame print("Original DataFrame:", df, sep='\n') # Modify a subset of the DataFrame using iloc df.iloc[1:3, 0] = ['x', 'y'] # Display the modified DataFrame print('Modified DataFrame:',df, sep='\n')
以上代码的输出如下:
Original DataFrame: col1 col2 0 a b 1 c d 2 e f 3 g h Modified DataFrame: col1 col2 0 a b 1 x d 2 y f 3 g h