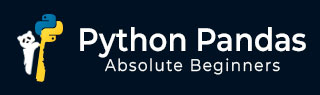
- Python Pandas 教程
- Python Pandas - 首页
- Python Pandas - 简介
- Python Pandas - 环境设置
- Python Pandas - 基础
- Python Pandas - 数据结构介绍
- Python Pandas - 索引对象
- Python Pandas - 面板 (Panel)
- Python Pandas - 基本功能
- Python Pandas - 索引和数据选择
- Python Pandas - Series
- Python Pandas - Series
- Python Pandas - 切片 Series 对象
- Python Pandas - Series 对象的属性
- Python Pandas - Series 对象上的算术运算
- Python Pandas - 将 Series 转换为其他对象
- Python Pandas - DataFrame
- Python Pandas - DataFrame
- Python Pandas - 访问 DataFrame
- Python Pandas - 切片 DataFrame 对象
- Python Pandas - 修改 DataFrame
- Python Pandas - 从 DataFrame 中删除行
- Python Pandas - DataFrame 上的算术运算
- Python Pandas - I/O 工具
- Python Pandas - I/O 工具
- Python Pandas - 使用 CSV 格式
- Python Pandas - 读取和写入 JSON 文件
- Python Pandas - 从 Excel 文件读取数据
- Python Pandas - 将数据写入 Excel 文件
- Python Pandas - 使用 HTML 数据
- Python Pandas - 剪贴板
- Python Pandas - 使用 HDF5 格式
- Python Pandas - 与 SQL 的比较
- Python Pandas - 数据处理
- Python Pandas - 排序
- Python Pandas - 重索引
- Python Pandas - 迭代
- Python Pandas - 连接
- Python Pandas - 统计函数
- Python Pandas - 描述性统计
- Python Pandas - 处理文本数据
- Python Pandas - 函数应用
- Python Pandas - 选项和自定义
- Python Pandas - 窗口函数
- Python Pandas - 聚合
- Python Pandas - 合并/连接
- Python Pandas - 多级索引
- Python Pandas - 多级索引基础
- Python Pandas - 使用多级索引进行索引
- Python Pandas - 使用多级索引进行高级重索引
- Python Pandas - 重命名多级索引标签
- Python Pandas - 对多级索引进行排序
- Python Pandas - 二元运算
- Python Pandas - 二元比较运算
- Python Pandas - 布尔索引
- Python Pandas - 布尔掩码
- Python Pandas - 数据重塑和透视表
- Python Pandas - 透视表
- Python Pandas - 堆叠和取消堆叠
- Python Pandas - 熔化
- Python Pandas - 计算虚拟变量
- Python Pandas - 分类数据
- Python Pandas - 分类数据
- Python Pandas - 分类数据的排序和排序
- Python Pandas - 分类数据的比较
- Python Pandas - 处理缺失数据
- Python Pandas - 缺失数据
- Python Pandas - 填充缺失数据
- Python Pandas - 缺失值的插值
- Python Pandas - 删除缺失数据
- Python Pandas - 使用缺失数据进行计算
- Python Pandas - 处理重复数据
- Python Pandas - 重复数据
- Python Pandas - 计数和检索唯一元素
- Python Pandas - 重复标签
- Python Pandas - 分组和聚合
- Python Pandas - GroupBy
- Python Pandas - 时间序列数据
- Python Pandas - 日期功能
- Python Pandas - 时间差 (Timedelta)
- Python Pandas - 稀疏数据结构
- Python Pandas - 稀疏数据
- Python Pandas - 可视化
- Python Pandas - 可视化
- Python Pandas - 其他概念
- Python Pandas - 注意事项和陷阱
- Python Pandas 有用资源
- Python Pandas - 快速指南
- Python Pandas - 有用资源
- Python Pandas - 讨论
Python Pandas - 修改 DataFrame
Pandas DataFrame 是一种二维数据结构,可用于以表格格式存储数据,它由行和列组成。修改 Pandas DataFrame 是数据操作和预处理中的一项基本任务。常见的修改包括重命名列或行标签、为附加数据添加或插入新列、更新或替换现有列的内容以及删除不需要的列。
在本教程中,我们将学习如何以不同的方式修改 Pandas DataFrame。
重命名 DataFrame 的列或行标签
Pandas 的 DataFrame.rename() 方法用于重命名 DataFrame 的一个或多个列或行标签。这在需要提高 DataFrame 数据的可读性的情况下非常有用。
示例:重命名 DataFrame 列标签
以下示例使用 DataFrame.rename() 方法重命名 DataFrame 的列名。
import pandas as pd # Create a DataFrame df = pd.DataFrame({'A': [1, 2, 3],'B': [4, 5, 6]}) # Display original DataFrame print("Original DataFrame:") print(df) # Rename column 'A' to 'aa' df = df.rename(columns={'A': 'aa'}) # Display modified DataFrame print("Modified DataFrame:") print(df)
以下是上述代码的输出 -
Original DataFrame: A B 0 1 4 1 2 5 2 3 6 Modified DataFrame: aa B 0 1 4 1 2 5 2 3 6
示例:重命名 DataFrame 行标签
与上述示例类似,这也会使用 DataFrame.rename() 方法重命名 DataFrame 的行标签。
import pandas as pd # Create a DataFrame df = pd.DataFrame({'A': [1, 2, 3],'B': [4, 5, 6]}, index=['x', 'y', 'z']) # Display original DataFrame print("Original DataFrame:") print(df) # Rename the multiple row labels df = df.rename(index={'x': 'r1', 'y':'r2', 'z':'r3'}) # Display modified DataFrame print("Modified DataFrame:") print(df)
以下是上述代码的输出 -
Original DataFrame: A B x 1 4 y 2 5 z 3 6 Modified DataFrame: A B r1 1 4 r2 2 5 r3 3 6
添加或插入列
向现有 DataFrame 添加新列非常简单。最简单的方法是使用新列名直接为 DataFrame 分配值。此外,您可以使用 DataFrame.insert() 方法在指定位置插入新列。
示例:直接添加新列
以下示例演示如何直接向 DataFrame 添加新列。
import pandas as pd # Create a DataFrame df = pd.DataFrame({'A': [1, 2, 3],'B': [4, 5, 6]}) # Add a new column 'C' with values df['C'] = [7, 8, 9] # Display updated DataFrame print("DataFrame after adding a new column 'C':") print(df)
以下是上述代码的输出 -
DataFrame after adding a new column 'C': A B C 0 1 4 7 1 2 5 8 2 3 6 9
示例:在特定位置插入列
此示例使用 DataFrame.insert() 方法在现有 DataFrame 的特定位置添加新列。
import pandas as pd # Create a DataFrame df = pd.DataFrame({'A': [1, 2, 3],'B': [4, 5, 6]}) # Insert a new column 'D' at position 1 df.insert(1, 'D', [10, 11, 12]) # Display updated DataFrame print("DataFrame after inserting column 'D' at position 1:") print(df)
以下是上述代码的输出 -
DataFrame after inserting column 'D' at position 1: A D B 0 1 10 4 1 2 11 5 2 3 12 6
替换 DataFrame 的内容
替换 DataFrame 的内容可以通过多种方式完成,其中一种最简单的方法是直接为 DataFrame 的特定部分分配新值。
示例:替换列的值
以下示例演示了用新数据替换特定列内容的最简单方法。
import pandas as pd # Create a DataFrame df = pd.DataFrame({'A': [1, 2, 3],'B': [4, 5, 6]}) # Replace the contents of column 'A' with new values df['A'] = [10, 20, 30] # Display updated DataFrame print("DataFrame after replacing column 'A':") print(df)
以下是上述代码的输出 -
DataFrame after replacing column 'A': A B 0 10 4 1 20 5 2 30 6
示例:使用 replace() 方法替换内容
您还可以使用 DataFrame.replace() 方法更改列中的特定值。此示例演示了相同的内容。
import pandas as pd # Create a DataFrame df = pd.DataFrame({'A': [1, 2, 3],'B': [4, 5, 6]}) # Display the Input DataFrame print("Original DataFrame:", df, sep='\n') # Replace the contents df.replace({'A': 1, 'B': 6}, 100, inplace=True) # Display updated DataFrame print("DataFrame after replacing column 'A':") print(df)
以下是上述代码的输出 -
Original DataFrame: A B 0 1 4 1 2 5 2 3 6 DataFrame after replacing column 'A': A B 0 100 4 1 2 5 2 3 100
删除列
删除 DataFrame 的单个或多个列可以使用 DataFrame.drop() 方法完成。
示例
以下是使用 DataFrame.drop() 方法从 Pandas DataFrame 中删除多个列的示例。
import pandas as pd # Create a DataFrame df = pd.DataFrame({'A': [1, 2, 3],'B': [4, 5, 6],'C': [7, 8, 9]}) # Display the original DataFrame print("Original DataFrame:", df, sep='\n') # Delete columns 'A' and 'B' df = df.drop(columns=['A', 'B']) # Display updated DataFrame print("DataFrame after deleting columns 'A' and 'B':") print(df)
以下是上述代码的输出 -
Original DataFrame: A B C 0 1 4 7 1 2 5 8 2 3 6 9 DataFrame after deleting columns 'A' and 'B': C 0 7 1 8 2 9