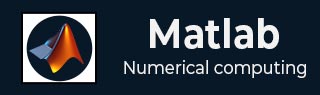
- Matlab 教程
- MATLAB - 首页
- MATLAB - 概述
- MATLAB - 功能
- MATLAB - 环境设置
- MATLAB - 编辑器
- MATLAB - 在线版
- MATLAB - 工作区
- MATLAB - 语法
- MATLAB - 变量
- MATLAB - 命令
- MATLAB - 数据类型
- MATLAB - 运算符
- MATLAB - 日期和时间
- MATLAB - 数字
- MATLAB - 随机数
- MATLAB - 字符串和字符
- MATLAB - 文本格式化
- MATLAB - 时间表
- MATLAB - M 文件
- MATLAB - 冒号表示法
- MATLAB - 数据导入
- MATLAB - 数据导出
- MATLAB - 数据归一化
- MATLAB - 预定义变量
- MATLAB - 决策
- MATLAB - 决策语句
- MATLAB - if 语句
- MATLAB - if else 语句
- MATLAB - if…elseif else 语句
- MATLAB - 嵌套 if 语句
- MATLAB - switch 语句
- MATLAB - 嵌套 switch
- MATLAB - 循环
- MATLAB - 循环
- MATLAB - for 循环
- MATLAB - while 循环
- MATLAB - 嵌套循环
- MATLAB - break 语句
- MATLAB - continue 语句
- MATLAB - end 语句
- MATLAB - 数组
- MATLAB - 数组
- MATLAB - 向量
- MATLAB - 转置运算符
- MATLAB - 数组索引
- MATLAB - 多维数组
- MATLAB - 兼容数组
- MATLAB - 分类数组
- MATLAB - 元胞数组
- MATLAB - 矩阵
- MATLAB - 稀疏矩阵
- MATLAB - 表格
- MATLAB - 结构体
- MATLAB - 数组乘法
- MATLAB - 数组除法
- MATLAB - 数组函数
- MATLAB - 函数
- MATLAB - 函数
- MATLAB - 函数参数
- MATLAB - 匿名函数
- MATLAB - 嵌套函数
- MATLAB - return 语句
- MATLAB - 空函数
- MATLAB - 局部函数
- MATLAB - 全局变量
- MATLAB - 函数句柄
- MATLAB - filter 函数
- MATLAB - 阶乘
- MATLAB - 私有函数
- MATLAB - 子函数
- MATLAB - 递归函数
- MATLAB - 函数优先级顺序
- MATLAB - map 函数
- MATLAB - mean 函数
- MATLAB - end 函数
- MATLAB - 错误处理
- MATLAB - 错误处理
- MATLAB - try...catch 语句
- MATLAB - 调试
- MATLAB - 绘图
- MATLAB - 绘图
- MATLAB - 绘制数组
- MATLAB - 绘制向量
- MATLAB - 条形图
- MATLAB - 直方图
- MATLAB - 图形
- MATLAB - 二维线图
- MATLAB - 三维图
- MATLAB - 图表格式化
- MATLAB - 对数坐标轴图
- MATLAB - 绘制误差条
- MATLAB - 绘制三维等高线图
- MATLAB - 极坐标图
- MATLAB - 散点图
- MATLAB - 绘制表达式或函数
- MATLAB - 绘制矩形
- MATLAB - 绘制频谱图
- MATLAB - 绘制网格曲面图
- MATLAB - 绘制正弦波
- MATLAB - 插值
- MATLAB - 插值
- MATLAB - 线性插值
- MATLAB - 二维数组插值
- MATLAB - 三维数组插值
- MATLAB - 多项式
- MATLAB - 多项式
- MATLAB - 多项式加法
- MATLAB - 多项式乘法
- MATLAB - 多项式除法
- MATLAB - 多项式的导数
- MATLAB - 变换
- MATLAB - 变换
- MATLAB - 拉普拉斯变换
- MATLAB - 拉普拉斯滤波器
- MATLAB - 高斯-拉普拉斯滤波器
- MATLAB - 逆傅里叶变换
- MATLAB - 傅里叶变换
- MATLAB - 快速傅里叶变换
- MATLAB - 二维逆余弦变换
- MATLAB - 向坐标轴添加图例
- MATLAB - 面向对象
- MATLAB - 面向对象编程
- MATLAB - 类和对象
- MATLAB - 函数重载
- MATLAB - 运算符重载
- MATLAB - 用户定义类
- MATLAB - 复制对象
- MATLAB - 代数
- MATLAB - 线性代数
- MATLAB - 高斯消去法
- MATLAB - 高斯-约旦消去法
- MATLAB - 行简化阶梯形矩阵
- MATLAB - 特征值和特征向量
- MATLAB - 积分
- MATLAB - 积分
- MATLAB - 二重积分
- MATLAB - 梯形法则
- MATLAB - 辛普森法则
- MATLAB - 其他
- MATLAB - 微积分
- MATLAB - 微分
- MATLAB - 矩阵的逆
- MATLAB - GNU Octave
- MATLAB - Simulink
- MATLAB - 有用资源
- MATLAB - 快速指南
- MATLAB - 有用资源
- MATLAB - 讨论
MATLAB - 行简化阶梯形矩阵
行简化阶梯形矩阵 (RREF) 是线性代数中使用的矩阵的一种特定形式,用于求解线性方程组、进行秩计算以及分析线性变换。矩阵的 RREF 是唯一的,并且可以通过一系列初等行运算获得:行交换、行缩放以及将一行乘以一个数加到另一行。
RREF 的定义
如果矩阵满足以下条件,则该矩阵处于行简化阶梯形。
- 主元为 1 - 每一非零行的首个非零元素为 1。
- 主元上方和下方为 0 - 每个主元 1 是其所在列中唯一的非零元素。
- 行排序 - 任何一行的主元都位于其上一行主元的右边。
- 零行 - 任何仅包含零的列都位于矩阵的底部。
让我们考虑一个简单的例子
假设我们有一个矩阵 A:
$$\mathrm{A \: = \: \begin{bmatrix} 1 & 2 & 1 \\ 2 & 4 & -3 \\ 3 & 6 & 4 \end{bmatrix}}$$
要找到它的 RREF,请执行以下步骤。
步骤 1 - 将第一行转换为具有主元 1(如有必要,除以主系数)。
R1 = [1 2 1].
步骤 2 - 通过从后续行中减去第一行的倍数来消除第一列中主元 1 下方的元素。
$$\mathrm{R2\:=\:\begin{bmatrix}2&4&-3\end{bmatrix}\:-2\:\times\:\begin{bmatrix}1&2&1\end{bmatrix}\:=\:\begin{bmatrix}0&0&-5\end{bmatrix}}$$
$$\mathrm{R3\:=\:\begin{bmatrix}3&6&4\end{bmatrix}\:-3\:\times\:\begin{bmatrix}1&2&1\end{bmatrix}\:=\:\begin{bmatrix}0&0&1\end{bmatrix}} $$
步骤 3 - 将新行转换为具有主元 1。
R3 = [ 0 0 1 ]
步骤 4 - 通过减去第三行的适当倍数来消除第三列中主元 1 上方的元素。
$$\mathrm{R1\:=\:\begin{bmatrix}1&2&1\end{bmatrix}\:-1\:\times\:\begin{bmatrix}0&0&1\end{bmatrix}\:=\:\begin{bmatrix}1&2&0\end{bmatrix}}$$
$$\mathrm{R2\:=\:\begin{bmatrix}0&0&-5\end{bmatrix}\:-(-5)\:\times\:\begin{bmatrix}0&0&1\end{bmatrix}\:=\:\begin{bmatrix}0&0&0\end{bmatrix}}$$
得到的 RREF 矩阵为:
$$\mathrm{R \: = \: \begin{bmatrix} 1 & 2 & 0 \\ 0 & 0 & 0 \\ 0 & 0 & 1 \end{bmatrix}}$$
使用 Matlab 计算行简化阶梯形矩阵
在 MATLAB 中,可以使用内置函数 rref() 来计算矩阵的行简化阶梯形矩阵 (RREF)。RREF 是经过行约简达到简化形式的矩阵,这使得求解线性方程组更加容易。
语法
R = rref(A) R = rref(A,tol) [R,p] = rref(A)
语法解释
R = rref(A) 使用高斯-约旦消元法给出矩阵 A 的简化版本,该方法包括一个步骤,通过在需要时交换行来确保计算更稳定。
R = rref(A, tol) 允许您设置容差级别 (tol),这有助于算法确定哪些列太小而不足以被认为重要。
行简化阶梯形矩阵 Matlab 示例
以下是一些演示如何有效使用此函数的示例:
示例 1
考虑以下线性方程组。
2x+4y = 10; 4x+9y = 19;
对于上述方程,我们
定义增广矩阵
A = [2 4 10; 4 9 19];
此矩阵表示方程组,其中前两列表示 x 和 y 的系数,最后一列表示方程右侧的常数。
使用 rref() 简化矩阵
R = rref(A);
rref() 函数将高斯-约旦消元法应用于矩阵 A。它通过使对角元素为 1 并使这些列中的所有其他元素为 0 来简化矩阵。
如果需要,算法将交换行以确保数值稳定性。这确保了在处理小数或大数时,计算不会出现舍入误差或不稳定性。
disp('The Reduced Row Echelon Form is:'); disp(R);
完整的代码如下所示:
% Define the augmented matrix A = [2 4 10; 4 9 19]; % Compute the Reduced Row Echelon Form R = rref(A); % Display the result disp('The Reduced Row Echelon Form is:'); disp(R);
执行后,得到的输出如下:
>> % Define the augmented matrix A = [2 4 10; 4 9 19]; % Compute the Reduced Row Echelon Form R = rref(A); % Display the result disp('The Reduced Row Echelon Form is:'); disp(R); The Reduced Row Echelon Form is: 1 0 7 0 1 -1 >>
该矩阵现在处于简化形式,称为行简化阶梯形矩阵 (RREF)。
线性方程的解为 x=7 和 y=-1
示例 2
考虑以下矩阵。
$$\mathrm{A \: = \: \begin{bmatrix} 1 & 2 & 3 \\ 2 & 4 & 6.0001 \\ 3 & 6 & 9 \end{bmatrix}}$$
此矩阵有一行几乎是其他行的线性组合,除了一个很小的差异(6 对 6.0001)。使用容差参数将允许我们决定是否应该忽略或考虑该小差异。
定义矩阵
A = [1 2 3; 2 4 6.0001; 3 6 9];
此矩阵几乎是秩亏的,因为第二行和第三行几乎是第一行的线性组合。
使用具有指定容差的 rref()
tol = 0.01; % Set a higher tolerance R_high_tol = rref(A, tol);
在这里,我们使用 0.01 的容差,这意味着任何小于 0.01 的值都将被视为零。在这种情况下,6 和 6.0001 之间的微小差异将被忽略。
tol = 1e-6; % Set a lower tolerance R_low_tol = rref(A, tol);
使用更小的容差 (1e-6),算法将认为即使是微小的差异也很重要,因此 6 和 6.0001 之间的微小差异将被考虑在内。
最终代码如下:
% Define the matrix A = [1 2 3; 2 4 6.0001; 3 6 9]; % Compute RREF with high tolerance tol = 0.01; R_high_tol = rref(A, tol); % Compute RREF with low tolerance tol = 1e-6; R_low_tol = rref(A, tol); % Display the results disp('Reduced Row Echelon Form with high tolerance:'); disp(R_high_tol); disp('Reduced Row Echelon Form with low tolerance:'); disp(R_low_tol);
执行后,得到的输出为:
>> % Define the matrix A = [1 2 3; 2 4 6.0001; 3 6 9]; % Compute RREF with high tolerance tol = 0.01; R_high_tol = rref(A, tol); % Compute RREF with low tolerance tol = 1e-6; R_low_tol = rref(A, tol); % Display the results disp('Reduced Row Echelon Form with high tolerance:'); disp(R_high_tol); disp('Reduced Row Echelon Form with low tolerance:'); disp(R_low_tol); Reduced Row Echelon Form with high tolerance: 1 2 3 0 0 0 0 0 0 Reduced Row Echelon Form with low tolerance: 1 2 0 0 0 1 0 0 0 >>