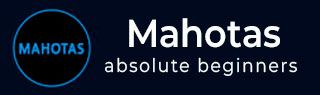
- Mahotas 教程
- Mahotas - 首页
- Mahotas - 简介
- Mahotas - 计算机视觉
- Mahotas - 历史
- Mahotas - 特性
- Mahotas - 安装
- Mahotas 处理图像
- Mahotas - 处理图像
- Mahotas - 加载图像
- Mahotas - 加载灰度图像
- Mahotas - 显示图像
- Mahotas - 显示图像形状
- Mahotas - 保存图像
- Mahotas - 图像的质心
- Mahotas - 图像卷积
- Mahotas - 创建 RGB 图像
- Mahotas - 图像的欧拉数
- Mahotas - 图像中零值的比例
- Mahotas - 获取图像矩
- Mahotas - 图像中的局部最大值
- Mahotas - 图像椭圆轴
- Mahotas - 图像拉伸 RGB
- Mahotas 颜色空间转换
- Mahotas - 颜色空间转换
- Mahotas - RGB 到灰度转换
- Mahotas - RGB 到 LAB 转换
- Mahotas - RGB 到 Sepia 转换
- Mahotas - RGB 到 XYZ 转换
- Mahotas - XYZ 到 LAB 转换
- Mahotas - XYZ 到 RGB 转换
- Mahotas - 增加伽马校正
- Mahotas - 拉伸伽马校正
- Mahotas 标记图像函数
- Mahotas - 标记图像函数
- Mahotas - 标记图像
- Mahotas - 过滤区域
- Mahotas - 边界像素
- Mahotas - 形态学操作
- Mahotas - 形态学算子
- Mahotas - 查找图像均值
- Mahotas - 裁剪图像
- Mahotas - 图像的偏心率
- Mahotas - 叠加图像
- Mahotas - 图像的圆度
- Mahotas - 调整图像大小
- Mahotas - 图像的直方图
- Mahotas - 膨胀图像
- Mahotas - 腐蚀图像
- Mahotas - 分水岭算法
- Mahotas - 图像的开运算
- Mahotas - 图像的闭运算
- Mahotas - 填充图像中的孔洞
- Mahotas - 条件膨胀图像
- Mahotas - 条件腐蚀图像
- Mahotas - 图像的条件分水岭算法
- Mahotas - 图像中的局部最小值
- Mahotas - 图像的区域最大值
- Mahotas - 图像的区域最小值
- Mahotas - 高级概念
- Mahotas - 图像阈值化
- Mahotas - 设置阈值
- Mahotas - 软阈值
- Mahotas - Bernsen 局部阈值化
- Mahotas - 小波变换
- 制作图像小波中心
- Mahotas - 距离变换
- Mahotas - 多边形实用程序
- Mahotas - 局部二值模式
- 阈值邻域统计
- Mahotas - Haralic 特征
- 标记区域的权重
- Mahotas - Zernike 特征
- Mahotas - Zernike 矩
- Mahotas - 排序滤波器
- Mahotas - 2D 拉普拉斯滤波器
- Mahotas - 多数滤波器
- Mahotas - 均值滤波器
- Mahotas - 中值滤波器
- Mahotas - Otsu 方法
- Mahotas - 高斯滤波
- Mahotas - 击中与不击中变换
- Mahotas - 标记最大数组
- Mahotas - 图像的平均值
- Mahotas - SURF 密集点
- Mahotas - SURF 积分
- Mahotas - Haar 变换
- 突出显示图像最大值
- 计算线性二值模式
- 获取标签的边界
- 反转 Haar 变换
- Riddler-Calvard 方法
- 标记区域的大小
- Mahotas - 模板匹配
- 加速鲁棒特征
- 删除带边框的标记
- Mahotas - Daubechies 小波
- Mahotas - Sobel 边缘检测
Mahotas - 计算线性二值模式
线性二值模式 (LBP) 用于分析图像中的模式。它将图像中中心像素的强度值与其相邻像素进行比较,并将结果编码成二进制模式(0 或 1)。
想象一下,您有一幅灰度图像,其中每个像素代表从黑色到白色的灰色阴影。LBP 将图像划分为小区域。
对于每个区域,它都会查看中心像素并将其亮度与相邻像素进行比较。
如果相邻像素的亮度高于或等于中心像素,则将其分配值为 1;否则,将其分配值为 0。此过程对所有相邻像素重复,从而创建二进制模式。
在 Mahotas 中计算线性二值模式
在 Mahotas 中,我们可以使用 **features.lbp()** 函数计算图像中的线性二值模式。该函数将中心像素的亮度与其邻居进行比较,并根据比较结果分配二进制值(0 或 1)。
然后将这些二进制值组合起来,创建描述每个区域纹理的二进制模式。通过对所有区域执行此操作,将创建一个直方图以统计图像中每个模式的出现次数。
直方图有助于我们了解图像中纹理的分布。
mahotas.features.lbp() 函数
mahotas.features.lbp() 函数将灰度图像作为输入,并返回每个像素的二进制值。然后使用二进制值创建线性二值模式的直方图。
直方图的 x 轴表示计算出的 LBP 值,而 y 轴表示 LBP 值的频率。
语法
以下是 mahotas 中 lbp() 函数的基本语法:
mahotas.features.lbp(image, radius, points, ignore_zeros=False)
其中,
**image** - 输入灰度图像。
**radius** - 指定用于比较像素强度的区域的大小。
**points** - 确定计算每个像素的 LBP 时应考虑的相邻像素数量。
**ignore_zeros (可选)** - 一个标志,指定是否忽略零值像素(默认值为 false)。
示例
在以下示例中,我们使用 mh.features.lbp() 函数计算线性二值模式。
import mahotas as mh import numpy as np import matplotlib.pyplot as mtplt # Loading the image image = mh.imread('nature.jpeg') # Converting it to grayscale image = mh.colors.rgb2gray(image) # Computing linear binary patterns lbp = mh.features.lbp(image, 5, 5) # Creating a figure and axes for subplots fig, axes = mtplt.subplots(1, 2) # Displaying the original image axes[0].imshow(image, cmap='gray') axes[0].set_title('Original Image') axes[0].set_axis_off() # Displaying the linear binary patterns axes[1].hist(lbp) axes[1].set_title('Linear Binary Patterns') axes[1].set_xlabel('LBP Value') axes[1].set_ylabel('Frequency') # Adjusting spacing between subplots mtplt.tight_layout() # Showing the figures mtplt.show()
输出
以下是上述代码的输出:
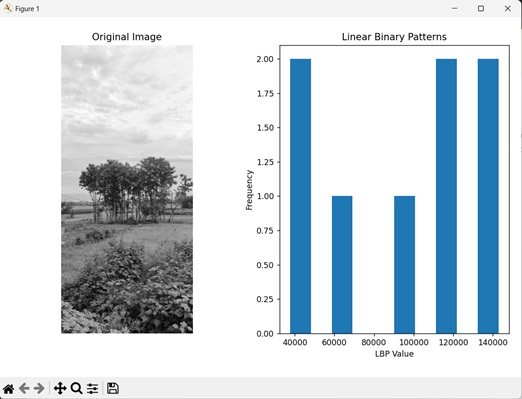
忽略零值像素
在计算线性二值模式时,我们可以忽略零值像素。零值像素是指强度值为 0 的像素。
它们通常表示图像的背景,但也可能表示噪声。在灰度图像中,零值像素由颜色“黑色”表示。
在 mahotas 中,我们可以将 **ignore_zeros** 参数设置为布尔值“True”以在 mh.features.lbp() 函数中排除零值像素。
示例
以下示例显示了通过忽略零值像素计算线性二值模式。
import mahotas as mh import numpy as np import matplotlib.pyplot as mtplt # Loading the image image = mh.imread('sea.bmp') # Converting it to grayscale image = mh.colors.rgb2gray(image) # Computing linear binary patterns lbp = mh.features.lbp(image, 20, 10, ignore_zeros=True) # Creating a figure and axes for subplots fig, axes = mtplt.subplots(1, 2) # Displaying the original image axes[0].imshow(image, cmap='gray') axes[0].set_title('Original Image') axes[0].set_axis_off() # Displaying the linear binary patterns axes[1].hist(lbp) axes[1].set_title('Linear Binary Patterns') axes[1].set_xlabel('LBP Value') axes[1].set_ylabel('Frequency') # Adjusting spacing between subplots mtplt.tight_layout() # Showing the figures mtplt.show()
输出
上述代码的输出如下:
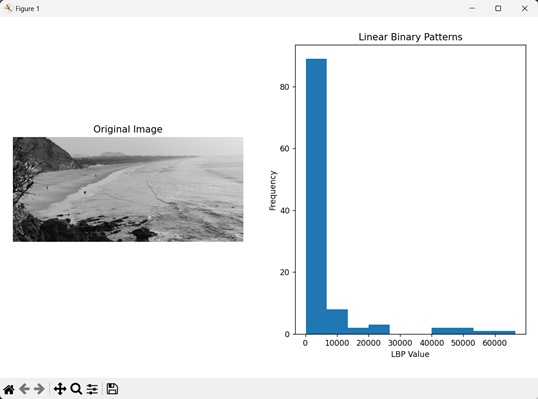
特定区域的 LBP
我们还可以计算图像中特定区域的线性二值模式。特定区域是指具有任何尺寸的图像的一部分。可以通过裁剪原始图像获得。
在 mahotas 中,要计算特定区域的线性二值模式,我们首先需要从图像中找到感兴趣的区域。为此,我们分别为 x 和 y 坐标指定起始和结束像素值。然后,我们可以使用 lbp() 函数计算该区域的 LBP。
例如,如果我们将值指定为 [300:800],则该区域将从第 300 个像素开始,并在垂直方向(y 轴)上一直延伸到第 800 个像素。
示例
这里,我们正在计算指定灰度图像的特定部分的 LBP。
import mahotas as mh import numpy as np import matplotlib.pyplot as mtplt # Loading the image image = mh.imread('tree.tiff') # Converting it to grayscale image = mh.colors.rgb2gray(image) # Specifying a region of interest image = image[300:800] # Computing linear binary patterns lbp = mh.features.lbp(image, 20, 10) # Creating a figure and axes for subplots fig, axes = mtplt.subplots(1, 2) # Displaying the original image axes[0].imshow(image, cmap='gray') axes[0].set_title('Original Image') axes[0].set_axis_off() # Displaying the linear binary patterns axes[1].hist(lbp) axes[1].set_title('Linear Binary Patterns') axes[1].set_xlabel('LBP Value') axes[1].set_ylabel('Frequency') # Adjusting spacing between subplots mtplt.tight_layout() # Showing the figures mtplt.show()
输出
执行上述代码后,我们将获得以下输出:
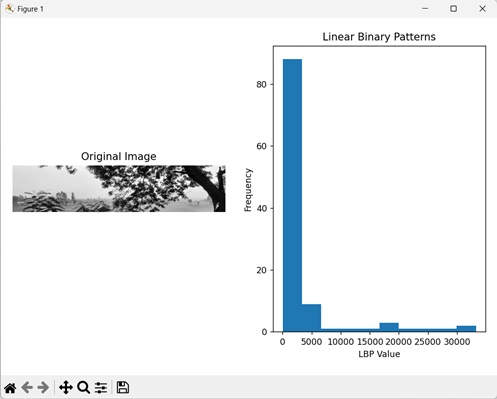