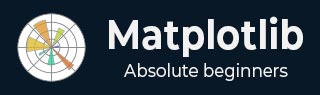
- Matplotlib基础
- Matplotlib - 首页
- Matplotlib - 简介
- Matplotlib - 与Seaborn对比
- Matplotlib - 环境搭建
- Matplotlib - Anaconda发行版
- Matplotlib - Jupyter Notebook
- Matplotlib - Pyplot API
- Matplotlib - 简单绘图
- Matplotlib - 保存图片
- Matplotlib - 标记
- Matplotlib - 图形
- Matplotlib - 样式
- Matplotlib - 图例
- Matplotlib - 颜色
- Matplotlib - 颜色图
- Matplotlib - 颜色图归一化
- Matplotlib - 选择颜色图
- Matplotlib - 颜色条
- Matplotlib - 文本
- Matplotlib - 文本属性
- Matplotlib - 子图标题
- Matplotlib - 图片
- Matplotlib - 图片蒙版
- Matplotlib - 注释
- Matplotlib - 箭头
- Matplotlib - 字体
- Matplotlib - 什么是字体?
- 全局设置字体属性
- Matplotlib - 字体索引
- Matplotlib - 字体属性
- Matplotlib - 比例尺
- Matplotlib - 线性与对数比例尺
- Matplotlib - 对称对数和logit比例尺
- Matplotlib - LaTeX
- Matplotlib - 什么是LaTeX?
- Matplotlib - LaTeX用于数学表达式
- Matplotlib - 在注释中使用LaTeX文本格式
- Matplotlib - PostScript
- 启用注释中的LaTex渲染
- Matplotlib - 数学表达式
- Matplotlib - 动画
- Matplotlib - 绘图元素
- Matplotlib - 使用Cycler进行样式设置
- Matplotlib - 路径
- Matplotlib - 路径效果
- Matplotlib - 变换
- Matplotlib - 刻度和刻度标签
- Matplotlib - 弧度刻度
- Matplotlib - 日期刻度
- Matplotlib - 刻度格式化器
- Matplotlib - 刻度定位器
- Matplotlib - 基本单位
- Matplotlib - 自动缩放
- Matplotlib - 反转坐标轴
- Matplotlib - 对数坐标轴
- Matplotlib - Symlog
- Matplotlib - 单位处理
- Matplotlib - 带单位的椭圆
- Matplotlib - 脊柱
- Matplotlib - 坐标轴范围
- Matplotlib - 坐标轴比例尺
- Matplotlib - 坐标轴刻度
- Matplotlib - 坐标轴格式化
- Matplotlib - Axes类
- Matplotlib - 双坐标轴
- Matplotlib - Figure类
- Matplotlib - 多图
- Matplotlib - 网格
- Matplotlib - 面向对象接口
- Matplotlib - PyLab模块
- Matplotlib - Subplots()函数
- Matplotlib - Subplot2grid()函数
- Matplotlib - 固定位置的绘图元素
- Matplotlib - 手动等高线
- Matplotlib - 坐标报告
- Matplotlib - AGG过滤器
- Matplotlib - 飘带框
- Matplotlib - 填充螺旋线
- Matplotlib - Findobj演示
- Matplotlib - 超链接
- Matplotlib - 图片缩略图
- Matplotlib - 使用关键字绘图
- Matplotlib - 创建Logo
- Matplotlib - 多页PDF
- Matplotlib - 多进程
- Matplotlib - 打印标准输出
- Matplotlib - 复合路径
- Matplotlib - Sankey类
- Matplotlib - MRI与EEG
- Matplotlib - 样式表
- Matplotlib - 背景颜色
- Matplotlib - Basemap
- Matplotlib事件处理
- Matplotlib - 事件处理
- Matplotlib - 关闭事件
- Matplotlib - 鼠标移动
- Matplotlib - 点击事件
- Matplotlib - 滚动事件
- Matplotlib - 按键事件
- Matplotlib - 选择事件
- Matplotlib - 哈哈镜
- Matplotlib - 路径编辑器
- Matplotlib - 多边形编辑器
- Matplotlib - 定时器
- Matplotlib - Viewlims
- Matplotlib - 缩放窗口
- Matplotlib部件
- Matplotlib - 游标部件
- Matplotlib - 带注释的游标
- Matplotlib - 按钮部件
- Matplotlib - 复选框
- Matplotlib - 套索选择器
- Matplotlib - 菜单部件
- Matplotlib - 鼠标游标
- Matplotlib - 多游标
- Matplotlib - 多边形选择器
- Matplotlib - 单选按钮
- Matplotlib - 范围滑块
- Matplotlib - 矩形选择器
- Matplotlib - 椭圆选择器
- Matplotlib - 滑块部件
- Matplotlib - 跨度选择器
- Matplotlib - 文本框
- Matplotlib绘图
- Matplotlib - 条形图
- Matplotlib - 直方图
- Matplotlib - 饼图
- Matplotlib - 散点图
- Matplotlib - 箱线图
- Matplotlib - 小提琴图
- Matplotlib - 等高线图
- Matplotlib - 3D绘图
- Matplotlib - 3D等高线
- Matplotlib - 3D线框图
- Matplotlib - 3D曲面图
- Matplotlib - 矢羽图
- Matplotlib有用资源
- Matplotlib - 快速指南
- Matplotlib - 有用资源
- Matplotlib - 讨论
Matplotlib - 3D曲面图
3D曲面图是一种可视化具有三个维度(长度、宽度和高度)的数据的方法。
想象一下一个带有丘陵和山谷的地形,曲面上的每个点都代表一个特定的值。在3D曲面图中,这些点绘制在三维空间中,形成一个曲面,显示数据如何在不同位置变化。这就像查看数据的三个维度地图,其中曲面的高度代表每个点的数据值。
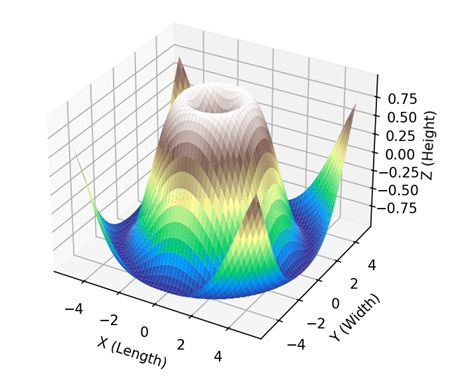
Matplotlib中的3D曲面图
在Matplotlib中,3D曲面图是多个点连接成类似图形的三维空间中的特定区域的视觉表示。我们可以使用“mpl_toolkits.mplot3d”模块中的plot_surface()函数在Matplotlib中创建3D曲面图。它将X、Y和Z坐标作为数组,并通过连接这三个坐标创建一个连续的图形。
让我们从绘制一个基本的3D曲面图开始。
基本的3D曲面图
Matplotlib中的基本3D曲面图是在三维空间中表示图形的一种方式,具有X、Y和Z轴。坐标形成一个曲面,其中每个点的海拔或深度(Z轴)赋予绘图其三维形状。
示例
在下面的示例中,我们通过均匀地间隔X和Y坐标来创建一个基本的3D曲面图,然后根据X和Y坐标的值找到Z坐标 -
import matplotlib.pyplot as plt import numpy as np from mpl_toolkits.mplot3d import Axes3D # Creating data x = np.linspace(-5, 5, 100) y = np.linspace(-5, 5, 100) X, Y = np.meshgrid(x, y) Z = np.sin(np.sqrt(X**2 + Y**2)) # Creating a 3D plot fig = plt.figure() ax = fig.add_subplot(111, projection='3d') # Plotting the basic 3D surface ax.plot_surface(X, Y, Z, cmap='viridis') # Customizing the plot ax.set_xlabel('X-axis') ax.set_ylabel('Y-axis') ax.set_zlabel('Z-axis') ax.set_title('Basic 3D Surface Plot') # Displaying the plot plt.show()
输出
以下是上述代码的输出 -
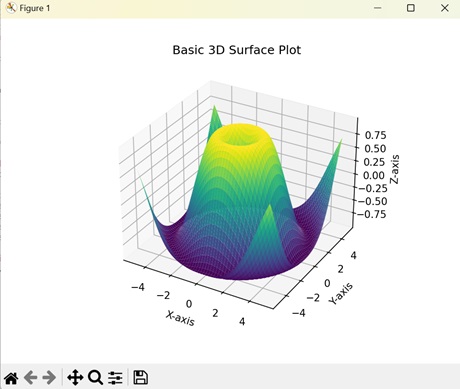
参数化3D曲面图
Matplotlib中的参数化3D曲面图使用数学方程在三维空间中定义图形。这些方程描述了X、Y和Z坐标的值如何随参数值的变化而变化。
示例
在这里,我们通过根据初始数据点(u, v)、大小(R)和厚度(r)参数化X、Y和Z坐标来创建一个参数化3D曲面图。生成的图显示了一个甜甜圈形状的曲面图 -
import matplotlib.pyplot as plt import numpy as np from mpl_toolkits.mplot3d import Axes3D # Parametric equations for a torus def torus_parametric(u, v, R=1, r=0.3): x = (R + r * np.cos(v)) * np.cos(u) y = (R + r * np.cos(v)) * np.sin(u) z = r * np.sin(v) return x, y, z # Generating data u = np.linspace(0, 2 * np.pi, 100) v = np.linspace(0, 2 * np.pi, 100) U, V = np.meshgrid(u, v) X, Y, Z = torus_parametric(U, V) # Creating a 3D plot fig = plt.figure() ax = fig.add_subplot(111, projection='3d') # Plotting the parametric 3D surface ax.plot_surface(X, Y, Z, cmap='plasma') # Customizing the plot ax.set_xlabel('X-axis') ax.set_ylabel('Y-axis') ax.set_zlabel('Z-axis') ax.set_title('Parametric 3D Surface Plot (Torus)') # Displaying the plot plt.show()
输出
执行上述代码后,我们将得到以下输出 -
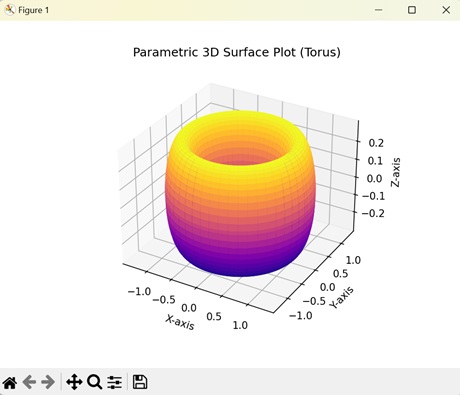
多个3D曲面图
在Matplotlib中,多个3D曲面图显示在三维空间中彼此堆叠的多个图形。每个图形都有X、Y和Z坐标的不同值。
示例
以下示例创建了两个彼此堆叠的3D曲面图。我们使用不同的方程来创建两个不同的3D曲面图。生成的图显示了在不同平面上具有不同颜色的两个曲面图 -
import matplotlib.pyplot as plt import numpy as np from mpl_toolkits.mplot3d import Axes3D # Creating data for two surfaces x = np.linspace(-5, 5, 100) y = np.linspace(-5, 5, 100) X, Y = np.meshgrid(x, y) Z1 = np.sin(np.sqrt(X**2 + Y**2)) Z2 = np.exp(-(X**2 + Y**2)) # Creating a 3D plot fig = plt.figure() ax = fig.add_subplot(111, projection='3d') # Plotting the two surfaces surf1 = ax.plot_surface(X, Y, Z1, cmap='viridis', alpha=0.7) surf2 = ax.plot_surface(X, Y, Z2, cmap='plasma', alpha=0.7) # Customize the plot ax.set_xlabel('X-axis') ax.set_ylabel('Y-axis') ax.set_zlabel('Z-axis') ax.set_title('Multiple Surfaces in 3D Surface Plot') # Adding a colorbar fig.colorbar(surf1, ax=ax, orientation='vertical', shrink=0.5, aspect=20) # Displaying the plot plt.show()
输出
执行上述代码后,我们将得到以下输出 -
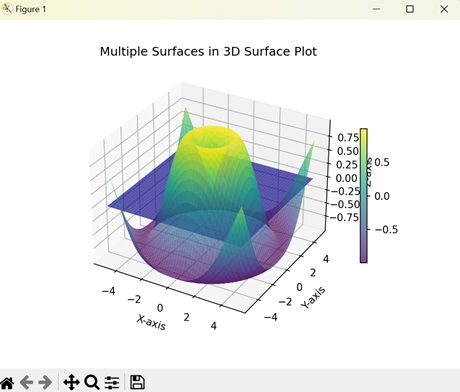
插值3D曲面图
Matplotlib中的插值3D曲面图帮助我们可视化X、Y和Z坐标随机分散的图形。插值有助于填充缺失的数据点以创建连续的图形。
示例
现在,我们正在创建一个插值3D曲面图。我们为X、Y和Z坐标生成随机值,然后使用线性插值来使用最近的数据点估计缺失数据点的值 -
import matplotlib.pyplot as plt import numpy as np from mpl_toolkits.mplot3d import Axes3D from scipy.interpolate import griddata # Creating irregularly spaced data np.random.seed(42) x = np.random.rand(100) y = np.random.rand(100) z = np.sin(x * y) # Creating a regular grid xi, yi = np.linspace(x.min(), x.max(), 100), np.linspace(y.min(), y.max(), 100) xi, yi = np.meshgrid(xi, yi) # Interpolating irregular data onto the regular grid zi = griddata((x, y), z, (xi, yi), method='linear') # Creating a 3D plot fig = plt.figure() ax = fig.add_subplot(111, projection='3d') # Plotting the 3D surface from irregular data using grid interpolation ax.plot_surface(xi, yi, zi, cmap='viridis', edgecolor='k') # Customizing the plot ax.set_xlabel('X-axis') ax.set_ylabel('Y-axis') ax.set_zlabel('Z-axis') ax.set_title('3D Surface Plot from Irregular Data (Grid Interpolation)') # Displaying the plot plt.show()
输出
执行上述代码后,我们将得到以下输出 -
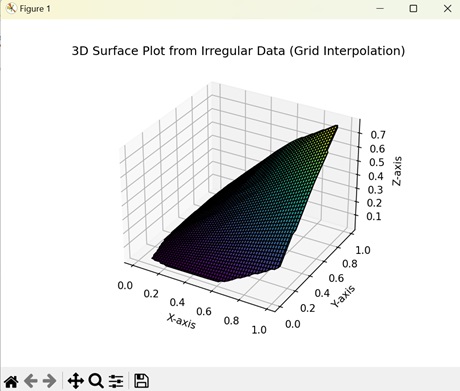